Using Spatial Analysis to Measure UK Gentrification
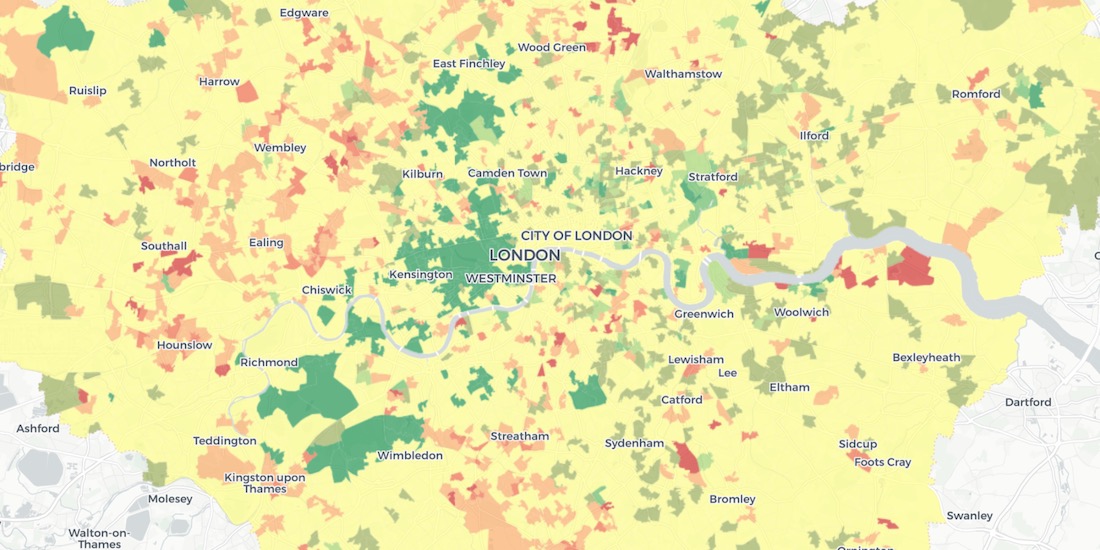
Deepening social inequalities have remained a constant in our contemporary urban landscapes. Particularly within global cities like London the disparities have become increasingly apparent with the city being home for the majority of the UK's richest 1% or indeed the 0.1% and also a large proportion of its urban poor. In spite of such polarisations a sense of indifference towards the needs of the average Londoner seemingly prevails throughout London's hyper-commodified housing market which is typified by sky-rocketing property prices and a chronic undersupply of truly affordable housing options.
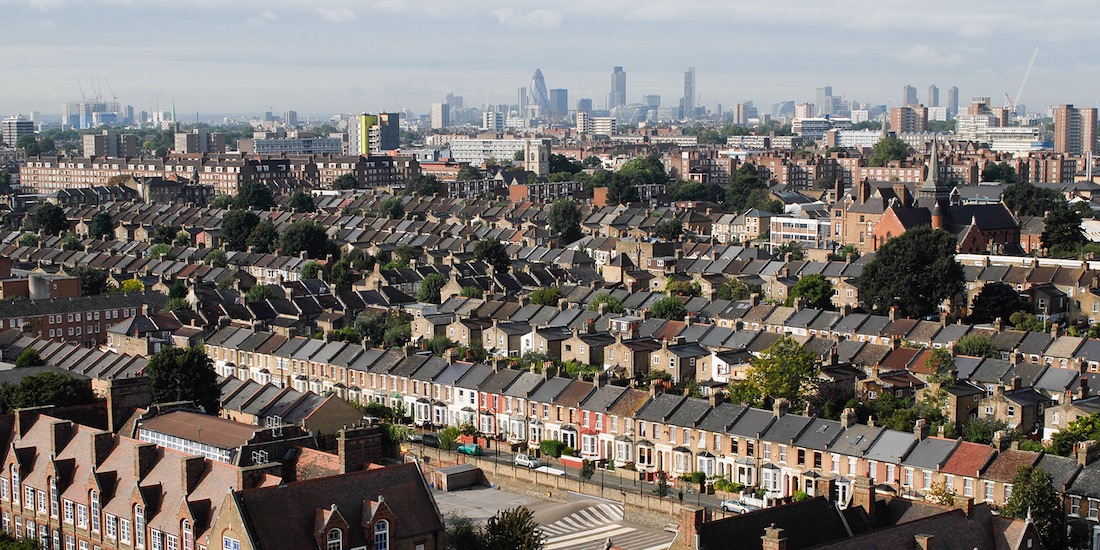
Gentrification emanating from the nexus of such trends has been endemic in London; propelling socio-spatial transformations in localised neighborhoods and displacing incumbent residents. Despite gentrification manifesting in more accentuated and diversified ways than ever before recent research has only been tepid in honing the critical edge necessary for effectively distinguishing gentrification from other forms of neighborhood change and to rigorously disaggregate gentrification's nuances.
Analysing & modeling Gentrification Trends
Addressing such issues the research team comprising Joshua Yee and Dr Adam Dennett from the Centre of Advanced Spatial Analysis (CASA) University College London (UCL) employed a novel empirical approach that synergised recent advancements in Machine Learning new data sets and Spatial Data Science techniques to systematically examine the variegated past and future trajectories of neighborhood change across London.
The nature of gentrification's mutations and its spatial patterning in London were further extracted using a combination of Principal Component Analysis (PCA) K-Means clustering and in-depth spatial analysis. Machine Learning (ML) was subsequently adopted to model gentrification's observed trends and predict its future frontiers; thereby offering policy-makers unprecedented and highly-contextualised insights into gentrification's projected dynamics and geographies.
The aims were threefold:
- Identify characterise and locate neighborhoods in London which have undergone recent gentrification specifically disaggregating the different types of change revealed by the data.
- Explore which neighborhoods are likely to be next in line.
- Present and make available data code and novel interactive visualisations as a comprehensive tool for supporting policy and decision making in the city.
Undergirding the originality of this study is a repertoire of novel ML spatial analytical techniques and new sources of multi-dimensional data leveraged to comprehensively examine the diversified patterns of neighborhood change and gentrification trajectories across London.
Data
The datasets utilised in this study were derived from three main sources:
- Office for National Statistics (ONS) in the form of data from the 2001 and 2011 Censuses (all harmonised to 2011 Geographies)
- Greater London Authority (GLA) in the form of the London Development Database containing digital records of all planning permissions over the same time period
- Consumer Data Research Centre (CDRC)'s estimates of population churn derived from electoral registers also for the same period.
Methodology
Diverse methods straddling across statistics ML and spatial analysis were chained into a multi-level workflow with every phase building upon results of the former in order to unpack the diversity and trajectories of gentrification across neighborhoods in London.
Firstly statistical geographies known as Lower Layer Super Output Areas (LSOAs) in London were classified according to whether they were ascending stable or declining in terms of house price levels of highly educated high socio-economic status residents or median income. Ascending neighborhoods were then further classified into gentrifying and non-gentrifying areas with three main typologies identified. The gentrification cluster was then further re-classified into three categories of gentrification. These classifications were then used to predict future areas of gentrification in the city using machine learning algorithms.
Results
Stage 1
Variables on house price levels of highly educated high socio-economic status residents or median income neighborhoods in London were combined into a composite index score using PCA and were then classified according to the changes observed between 2001 and 2011. Between 2001-2011 732 LSOAs were highlighted as ascending 619 LSOAs in decline whereas the remaining 3 484 LSOAs were considered stable. While LSOAs in ascent and decline were dotted throughout the city ascending LSOAs tended toward locations in Central and East London while a denser congregation of declining LSOAs was situated toward the western peripheries.
Stage 2
A k-means algorithm was used to isolate clusters of neighborhoods within the ascending areas with similar ascent characteristics. Three clusters were identified: Gentrification Incumbent-upgrading and re-urbanisation - their profiles shown in Figure 1 with their spatial distributions shown in Figure 2.
Figure 1: Clusters of Ascending Neighborhoods

Figure 2: Spatial Distribution of the Typologies of Neighborhood Ascent

Stage 3
Neighborhoods identified as gentrifying were further classified according to changes in the socio-economic status of residents house price and income. Three further clusters were identified: Super-gentrification Marginal Gentrification and Mainstream Gentrification (Figure 3 and 4).
Figure 3: Clusters of Gentrifying Neighborhoods

Figure 4: Spatial Distribution of Gentrification Types

Deeper spatial analysis identified statistically significant distinct hotspots of gentrifying neighborhoods and their variant typologies as visualised in the following map:
Stage 4
A suite of ML models were trained on the observed trends and spatial patterns of neighborhood ascent and gentrification that unfolded between 2001-2011 with the aim of predicting which LSOAs will gentrify in the near future and their corresponding typologies. To further optimise the model geographic covariates which layer on spatial perspectives and can enable the model to recognise the spatiality of gentrification's manifestations were incorporated in two ways. Firstly through the addition of an inner London dummy and secondly through a gentrification neighbor dummy. The addition of geographic covariates improved the prediction of gentrifying and non-gentrifying areas to 100% and 85% respectively.
A similar modeling methodology incorporating additional variables can be used to predict the specific typologies of future gentrifying LSOAs. According to the predictions visualized on the interactive map below super-gentrifying trends will potentially retain a stronghold over LSOAs in central-western London around Hampstead Heath Richmond Park and the northern edges of Barnet and Enfield. Previously isolated islands of super-gentrification near Chiswick Clapham South and Dulwich are nonetheless anticipated to expand. LSOAs experiencing marginal gentrification in future are likely to stay in East London although potentially becoming more extensive around Brockley and Kensal Green. Separately future mainstream forms of gentrification are predicted to be domineering within London's inner boroughs and north of the Thames in boroughs such as Camden Islington and Hackney.
Conclusions
The analysis has shown that two broad processes of neighborhood change – neighborhood ascent and decline – collectively accounted for 1 351 LSOAs or almost 30% of all LSOAs in the city. No borough except for the City of London was spared from these processes thereby underscoring the extensiveness of socio-spatial transformations happening in London.
Gentrifying LSOAs were found to comprise around 15% of all LSOAs in London; affecting over half-a-million residents. Percolating through traditionally richer upscale West London boroughs like Kensington and Chelsea and Westminster as well as boroughs in East London that are conventionally viewed as working-class and less desirable. These contrasting and indeed almost conflicting urban backdrops against which gentrification has materialised clearly hint toward its existence in variegated forms – to which our study has shown that super-gentrification marginal gentrification and mainstream gentrification were simultaneously taking place in London and are unlikely to diminish in the near future according to our model's predictions.
Want to discover more?