CUSTOMER STORIES
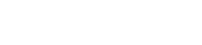
Applications of Geospatial Analysis within Fundamental Investment
Jefferies
Jefferies
,
The Client
The Challenge
Results
Why CARTO?
Transcription
This customer story has been adapted from a presentation given at the Spatial Data Science Conference 2019.
What is Equity Research?
Hello, thank you very much CARTO for having me here. There are some limits as to what I can share due to compliance in my organization and note that these are my views and not those of Jefferies.
We are using geospatial analysis to make actual investment decisions and advise our clients on them. Many of these are techniques that people assume are commonly applied and used throughout the industry. There’s a preconception around this, that is underutilized and underappreciated within the industry and something we are actively working to shift. So with that I want to give some context about equity research and why am I here.
My background is in risk management for a number of multi-manager hedge funds and I think it is important to incorporate that. Traditionally, data strategy, data research, was not formally a function within most of research. It was an afterthought, that was being done secondarily. In my previous role, we used a lot of data to self-evaluate and to analyze our teams to determine who was most effective. A lot of the skills I learned previously at being at a hedge fund were then translated, so instead of analyzing a portfolio and a group of investors, flip it to how can we actually look at forward-looking investment decisions and change behavior around how people think about this.
Another key thing I want to dig into is research. First let me set the stage for this so we’re on the same page: my teams are the people who fundamentally analyze a company. We’re not trying to build out a quantitative model to give us a framework to say we’re scoring a group of companies off the same metric, which is why this type of data is interesting. What we’re trying to do is find a key question that drives the key variable that a human is deciding is incredibly important, and shift it from being something that was previously assumed, to something that probably still has some assumption around it but can at least be based on a grounded fundamental observation.
Instead of: “I think more people are going to the store”, I’m using a dataset to actually tell me I know more people are going to this store. Another key piece around this was, the comments made around depth versus breadth. I assume that many people in the room are used to thinking about when you view the stock market, you view investing as a quantitative framework. Again, that is taking a single or a group of concepts applying to a large group of securities, and then, making some kind of signaling off of it to give you a group of names you want to own versus a group of names you don’t. That is not what we’re trying to do, we are trying to help this to understand a key question to be able to delve it apart.
How spatial analysis models are used in investment banking?
Another key piece around this that I want to touch on is what is Jefferies doing here? Why is this different? There are several other major players within this space that have functions not from the outside dissimilar to my own, with a high data component. Ten years ago, if you wanted to do that you couldn’t do it without having a significant number of people in the room working directly for you.
Now there are tools like CARTO and vendors like Safegraph who exist and we’re able to use them. So we’ve pivoted the way that we approach these problems to be closer to how another team would do it. Instead of focusing on assembling the information and beginning the process with questions such as “I need to go get GPS pings before I can even start my work” or “I need to understand every single POI or location for that particular retailer”, I can find someone else who’s very good at it, work with a tool like CARTO and have my team focus on the analysis and the outcome.
It gives us the opportunity to pivot the timeline that we do analysis. Historically, the work that I would be doing would take months or years to assemble. Now, we’re doing work in days and weeks. We’re not doing work yet in minutes and hours for the type of level of detail that I’ll talk about but we are doing it very quickly, which lets us react, and lets us advise our clients to things that are actively happening in the marketplace. This is also a variation, traditionally using “data” for these types of things was not utilized for that, because people thought you couldn’t source or utilize it fast enough, and now we can. I was just mentioning the structure of thinking about this, as assembling all this builds up the size of the team.
A part of what we do is focus on partnering with vendors to build tools that can then be reapplied, not just for ourselves but for our clients. A key thesis that I represent regularly is: if I’m able to draw a conclusion from this, my client will want to do that too because they want to check my work, make sure that they understand it, and be willing to put on a trade with it. They’re not simply going to take my output and trade 50 million dollars with it. They’re going to put the work into it, so as a result my relationship with vendors is such that I’m able to tell them I can use their data to answer a certain question. I would tell them we have to do more work to it, to use other tools, and to make other assumptions. But, by letting them be very good at assembling the POIs, understanding weather, physical location of stores, foot traffic and more, we can focus on the analysis for the key questions, as opposed to owning the entire flow of it. To do what I do now, if you started ten years ago that was required. It’s not necessary now, although you still have to be able to figure out who to work with and how to do it. That is a big piece of how we’ve been approaching the space and I also want to highlight that’s mirrored off of how we believe our clients are approaching the space.
Those kinds of tenants of how you approach this, where the value proposition starts and where it can be commoditized, versus where it can be reused, is an interesting piece that we’ve put a lot of effort into. I still focus on finding what the deep question is, that I need to work with, so that equivalency of some of the other conversations my clients or my internal research teams. I have a few hundred people covering a few thousand stocks, I let them tell me the thing they wish they needed to know, what is important to them, and we focus on how we can help them answer that, instead of building something from the beginning and hoping that it works.
Fundamental valuation: batting vs slugging
Within what I just described, I want to try to articulate why this is important to our clients. There are two key concepts within how a lot of people think about trading a portfolio without a fundamental valuation. We can use a baseball analogy to describe the two concepts: batting and slugging. Batting is when you put on 100 trades in a year: how many of them were you right? Slugging is: how much do you make on the ones that you make money on? How much do you lose on the ones that you lose money on?
I can’t change the behavior of batting, I cannot convince someone to buy a stock they weren’t going to necessarily buy. I can change behavior of slugging, I can convince someone that the data tells me that there’s actual grounded truth that makes you more comfortable in what you’re doing and makes you more confident in putting it on. Therefore, what I can do is to encourage my clients to be more profitable in the long term as they’re able to return higher amounts of the things they’re right on, because we’ve given them higher conviction in those things (assuming the data is correct). What this plays into is the difference between prediction and conviction. We’re not focused on prediction, I’m not trying to necessarily build a model that will out of the box predict something. I’m trying to give conviction into understanding deep questions and we found that using this type of data lends itself well to that.
People understand physical movement, people understand where things physically are. Generally if I look at every store and count how many people went there, that’s giving me a pretty good indication of sales. Statements like ‘the modern economy is offline, everything’s moving in that direction’ are necessarily true. There’s plenty of companies where we really care about it from that perspective. I care about the aggregate, pivot away from some of the other dialogues I’ve seen. I’m not trying to study individual behaviors, I’m trying to study the general trend and reaction to different things within the marketplace.
One of the key things we found a lot of success with is the ability to combine geospatial data. One can go and do this with other traditional data sets within finance. People know how to handle data like volume and price but it’s very hard to do that with a lot of other alternative datasets. It’s very challenging to connect actual transactions, back to incentive, or back to consumer behavior. But tying things back to a physical point in space is an understandable noble concept where if I combine time and space, I can perform analysis by looking at things like: weather combined with foot traffic or census information to give me demographic profiles. Applying that to make an investment decision, to say: “I, as a senior fundamental portfolio manager managing X dollars, understand this enough to use it, influence my behavior and will transact on it.” Which doesn’t make it good or bad but makes it something we’re able to capitalize on and use it as the first hook to get them to understand other things.
Real business use cases
We have actually utilized this tool to better understand relatively obvious things. For example it seems obvious that more people go to the music park Six Flags when it’s sunny but when you actually perform the analysis and prove it out, it tells the story and helps people understand it. By being able to isolate every single location, the weather patterns around those locations, aggregating them over time and across different places, highlighting the days when it rained and when it didn’t, we can articulate to our clients the ability to then help them better predict the future of that company. Because I can record the weather every day going forward, I now have a framework that helps me better understand this. It gives us the opportunity to help people better understand the long-term answer to their key questions.
Another one we’ve had a lot of success with, because it helps to show the cross relationship between online and offline, which is a good thing to articulate, is looking at delivery services such as Deliveroo, Delivery Hero, Grubhub & JustEat. All of these services work with physical locations but they all represent them in their own way. We have to cross relate them to figure out all 800,000 different restaurants that these different services work with. Which ones actually are only exclusively associated with one delivery company versus another? We can then be able to speak to if one delivery service is dominating a region of the United States versus another. Is one delivery service actually moving into the place that they said they were moving into? We can use tools like CARTO to help us understand the locations of these questions. Are they actually the same store, are they in the same area, is the demographic information useful, are people actually living in places where people are moving into? We can ask these questions instead of just accepting what the company is telling us about having more restaurants under their banner. We want to know if they actually have unique restaurants. Do they actually have restaurants that no one else has access to? Are they able to show that they own a market in a way that is not protected by somebody else?
We’ve also done significant analysis which would seem straightforward like store closures. Being able to help better understand if a store is closing, where the customer is going to go. These are traditionally done by just looking at how far away something is from something else. We can start to combine various datasets to look at historical behavior of the customers that went to the store that’s now closing, instead of simply assuming they’re going to go to the one down the street. Instead we can say they previously went to a different store, so therefore I can attribute how many customers were going to store A to store B accurately, instead of guessing at it.
This gives us the ability to help our clients understand how an event will occur and how it will actually play out. I think a key piece that I’m hitting at several times, is what we’re trying to do right now is not go out and tell people: “I have a prediction that tells you the answer to a question”. We’re using these types of tools to help us get a more general understanding of something that previously was relatively unknowable. When we historically looked at a company and asked them for their revenue we were limited to when they would tell us this information. Instead, we are trying to use these types of datasets and tools to help us better be able to articulate the actual events that are occurring within these types of companies and be able to help our clients understand them.
