CUSTOMER STORIES

Advancing Renewable Energy through Spatial Analysis and Visualization
NREL
NREL
,
The Client
The Challenge
Results
Why CARTO?
Transcription
Spatial Analysis for Renewable Energy
Today what I want to talk to you about is how the National Renewable Energy Laboratory (NREL) is using spatial analysis to advance renewable energy and visualization. NREL is the only federal laboratory in the United States that focuses solely on renewable energy, commercialization, development, and research. At NREL we really have a big focus on reliable, resilient and affordable clean energy. To get there we have to think big. We have to think about the big picture. This is how can we realize high penetrations of renewable energy, while simultaneously achieving these broad goals. Also what technologies help us get there, along with the constraints and challenges we are facing.
This visualization of the western and eastern interconnect of the United States and Canada is really putting that point across. . It’s basically showing every generator across that space on an hourly basis producing energy to meet our load. The point is that there’s a lot of data, it’s a very complex model, there’s a lot going on here, and there’s a lot that we have to think about. So, because these questions are so complex, we’ve been forced to develop state of the art tools, state of the art data, and state of the art visualizations that really help us dive into the complexities of these problems.
Really rooted in all of this and in some cases driving our results, in our understanding is spatial temporal modeling and data. In this gif here, you can see this is an immersive environment we have at NREL that allows us to get past even the 3D stage and start getting the in dimensional stage where we’re really exploring a wide parameter space.
There’s a fundamental challenge with renewable energy in that it’s variable and continuous in both space and time. This poses a lot of challenges to our traditional models. It comes down to how can you take something, a phenomena, that’s inherently continuous and variable, and cram it into discrete model space whether it’s nodal or regional. The biggest question is how can we ensure that we’re preserving and properly characterizing this resource?
Embracing Geospatial Data Science
It’s not just wind that has this issue or this challenge, it’s most of our renewable resources. They all have this geographic diversity and it’s actually a good challenge because it can also present reliability to our electric grid if we accurately represent it.
Now that we laid out the challenges, what is the solution? What can we apply to these challenges? Of course the answer is spatial analysis. When I say spatial analysis I’m not really referring to GIS. At NREL we’re moving beyond GIS. We’re embracing what we call geospatial data science. This is a combination of traditional GIS, geospatial big data, geo statistical analysis and spatial temporal and multivariate modeling and visualization.
At NREL, and in our spatial data science team, we have a broad research focus but it can be presented in this pyramid. This pyramid represents levels of potential where, as you move up the levels of the pyramid, you’re applying technological constraints or economical constraints to whittle down the resource until you ultimately find what the developable renewable energy potential is. Within each of those, you have research domains that we’re trying to look at: population dynamics, costs, wildlife issues, and barriers.
This is just a sample of what we’re looking at. But let’s look at more specifics. One of the big research focus areas where we’re advancing renewables is trying to understand barriers and regulations. What are the sociological, ecological and technological barriers preventing renewable energy from being deployed, and basically, what this comes down to very simply is we can’t and might not want to develop every wind slept ridge. There might be wildlife interactions that we want to prevent, there might be endangered species, or there might be a historical preservation that we want to keep in mind. The key here is that this fundamentally changes the available supply of renewable energy in a given region. As you can imagine, that’s pretty impactful.
Another way we’re using spatial analysis is through transmission analysis. Basically a lot of renewables are site dependent, you have to build on site and given that site dependent you have to evacuate that energy somehow and you have to do that through developing spur lines. Basically, what we’re trying to do with spatial analysis is understand a relationship between the quantity and quality of the renewable resource, and its proximity to existing infrastructure: how far do you have to go, what does that cost going to look like, could you actually do that given land development restrictions, and more.
Another example is through these wind farm characterizations in classification, it’s an archetype classification. Basically, we’re taking the existing wind turbine database throughout the entire United States and applying some characterizations to try to better understand existing development patterns. With this we can project development patterns out into the future, and what they might look like.
Renewable Energy Resource Assessment
Probably one of the most impactful pieces the geospatial data science team contributes to at NREL, is resource analysis. When I say resource it’s how much does the wind blow, how much does the sun shine, and I say it like that, but it’s actually really, really hard and there’re so many different models. There’s numerical weather prediction models, there’s semi empirical models, there’s physics based models. All have their differences, their accuracies, their deficiencies… What are those? When we compare them, when we use them in our downstream analysis and downstream the models how do they change our answers if they change it at all?
In addition, they have different accuracies. Can we compare these to ground measurements? They have different uncertainties, and they also have a variability. How do we understand the variability within the wind resource meaning that the sun doesn’t always shine the same, the wind doesn’t always blow the same. Year in and year out, you have El Niño effects, you have volcanoes erupt. How can these influence and impact our ability to generate energy, when we depend on the natural resources?
Probably, one of the funnest things we do at NREL is generator modeling, which is systems engineering combined with spatial analysis. What you’re seeing up here is generator performance of different photovoltaic technologies, and what we’re trying to understand is across broad geographies, how do they perform? After knowing how they perform, what are the relative economics, and what this allows us to do, is evaluate research and development pathways.
The Renewable Energy Potential (reV) Model
All of these are disparate pieces of analysis and research that we’re doing, and we’re starting to think about it a lot differently now. The way we’re thinking about is more of an ecosystem, a framework of analysis, because they all feed into one another. We’re doing this now with our spatial temporal techno-economic model called the Renewable Energy Potential Model or ‘reV’.
reV’ combines together all of what I was just talking about earlier. We have our resource information, systems engineering modeling, economics, technical spatial constraints, and our transmission analysis. We’re feeding this all together in this one ecosystem to come up with these geospatial supply curves to illuminate the results of technological advances, or constraints and barriers, whether they’re wildlife or they’re social, political, or more.
What’s really fascinating and what I get excited about as well, is that the spatial analysis and research we do, is illuminating. You can read a lot of research about what we do in it itself, but it also feeds into so much more. The results of the spatial analysis are fundamental and foundational to just about all downstream analysis when it comes to the electric grid, whether it’s unit commitment, whether it’s resource planning route distributed generation adoption, or policymaking. It all depends on spatial analysis which is pretty exciting.
As we become more successful at reducing the technological and economic barriers to renewable energy, we’re going to be faced with the changes to our built and our natural environment. So, what are those? How can we envision those? Can we anticipate those challenges? And if so, can we come up with different solutions?
Here I’m showing a modeling result projecting the growth of renewable energy out into 2050, the year 2050 under a different policy regime. You can see purple areas where we’re projecting wind farms to be developed and then we’re actually overlaying the existing turbines, and the yellow there that show a good alignment which proves two things. One, we’re doing a really good job of modeling and two, our industry is doing a really good job of developing cost optimal locations.
I want to thank CARTO for the fantastic job. It really helps get our research results out there. Sometimes research is not the prettiest thing, it gets put into a paper, gets lost in a journal. CARTO’s doing a fantastic job of helping us spread the word and getting our research out to the broader community, so thank you very much.

Want to make data-driven business predictions?
Related content

Endesa fulfilled a growing need for a location-based solution to improve site planning & demand modeling decisions. Discover how CARTO made it possible.
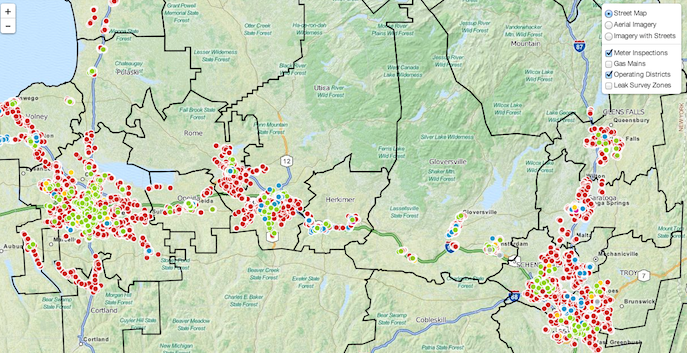
Learn how Premier Utility Services uses Location Intelligence to streamline large-scale gas infrastructure inspections, damage prevention, and leak detection.
.jpg)
Using Spatial Data Science enabled Skyhood to boost the performance of their mobile location services business.