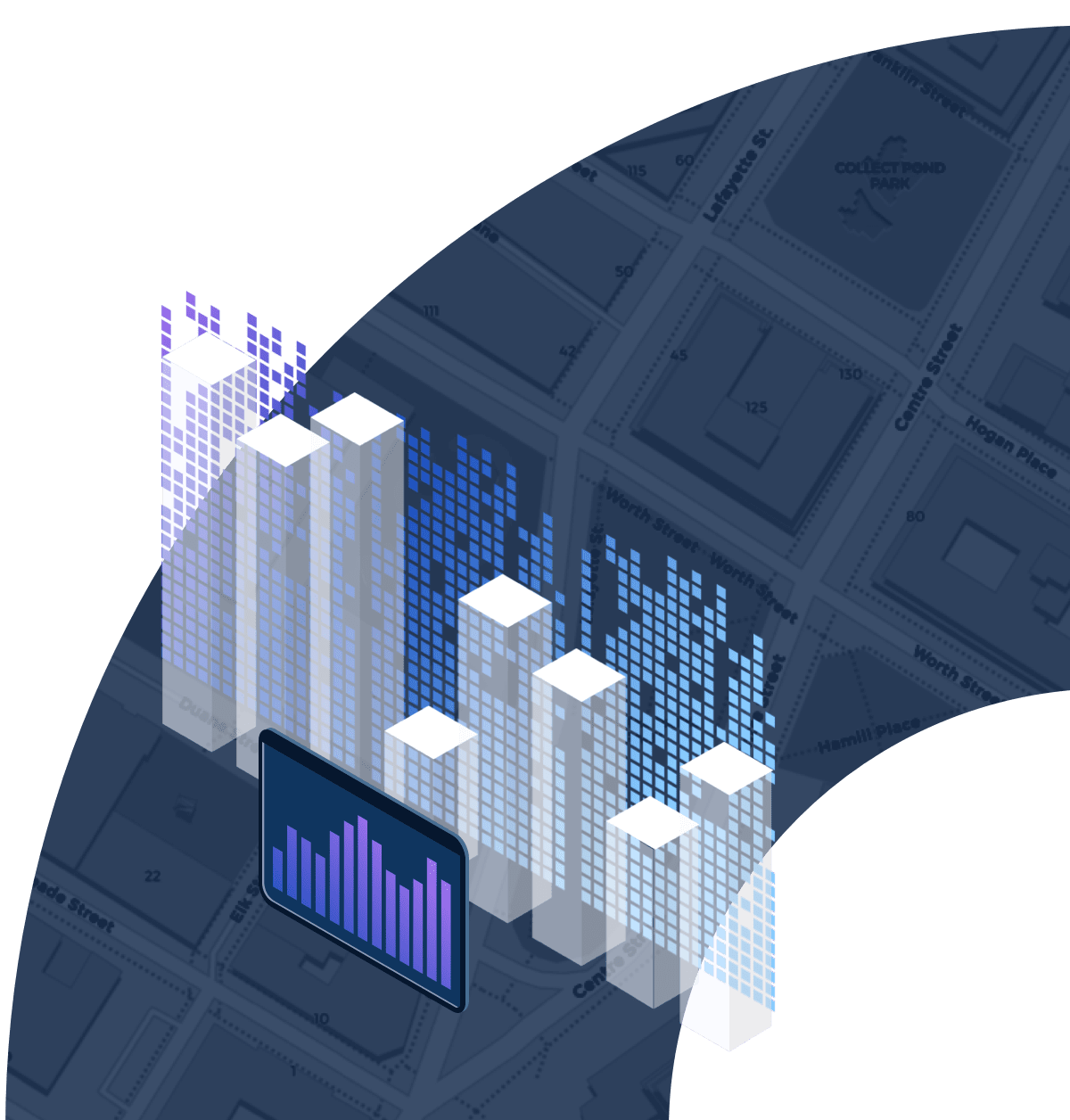
The go-to platform for Spatial Data Science
Only 1 in 3 Data Scientists have significant expertise working with location data. See why today's Spatial Data Scientist uses CARTO to speed up spatial analytics & visualization, natively in the cloud.
Try for freeSave time on "data admin"
Sourcing, evaluating & cleaning spatial data to enrich Data Science models typically take up 80% of your time, leaving only 20% of your time to focus on the most important part of the job - analysis. CARTO’s Data Observatory brings together thousands of location data streams such as human mobility, weather & demographics. Discover the most suitable datasets for your model, subscribe to them, select the variables you need & enrich your data all from your Jupyter notebook.
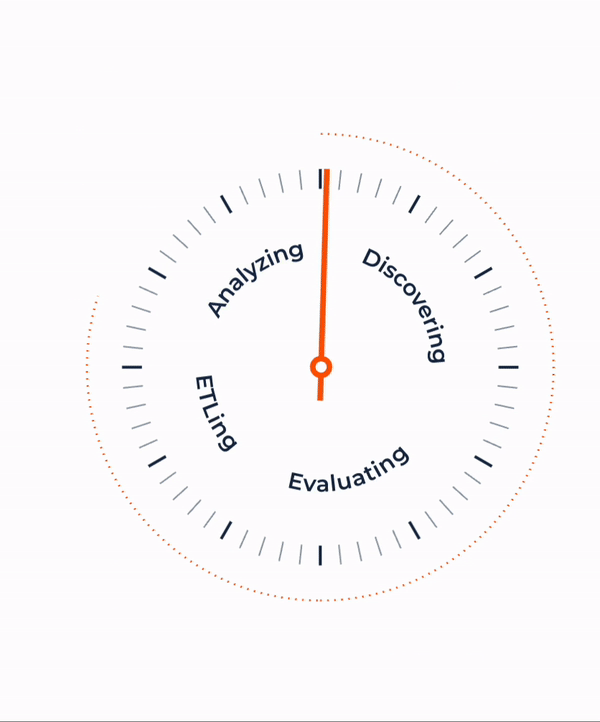
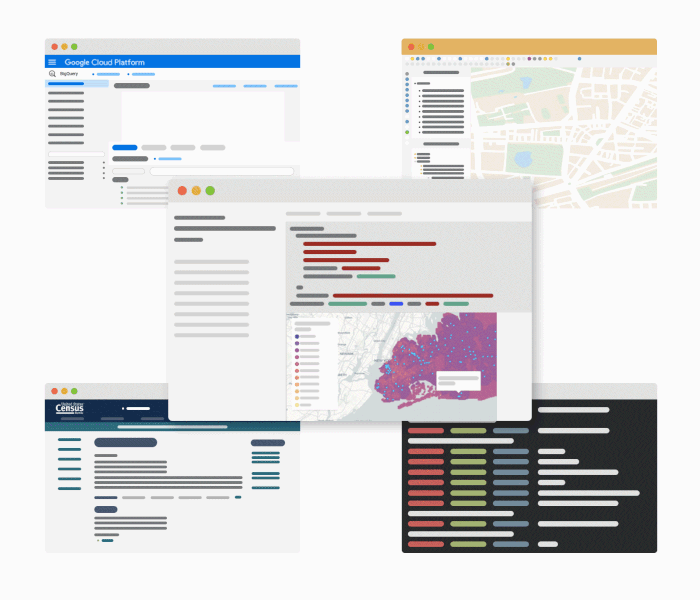
Less time context-switching, more time modeling
With our Analytics Toolbox you can execute advanced spatial analytics within your cloud data warehouse, and integrate maps & analysis in your current notebook environment. Visualize datasets straight out of Python notebooks, use location data services to convert plain text to geometries (geocoding), create areas of influence within a specific distance or travel time (isolines) & more. Once your analysis is done, add some pre-defined widgets and share the results — empowering business teams to understand & act on the insights from your models.
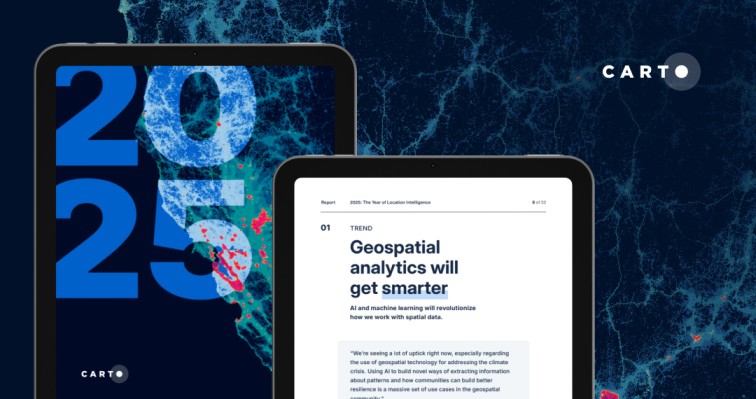
Seamless connectivity to your tools
Connect natively to the leading cloud data warehouses (such as Google BigQuery, Snowflake, Redshift & Databricks). Whether you’re looking for SaaS, private or public cloud or on-premise, our spatial analytics platform is ready to support location-based decisions on your terms.
