
What is Spatial Data Science?
Spatial Data Science (SDS) is a subset of Data Science that focuses on the special characteristics of spatial data, using modeling to know where and why things happen.
What is the meaning of Spatial Data Science?
Spatial data science (SDS) is a subset of Data Science that focuses on the unique characteristics of spatial data, moving beyond simply looking at where things happen to understand why they happen there.
SDS treats location, distance & spatial interactions as core aspects of the data using specialized methods & software to analyze, visualize & apply learnings to spatial use cases.

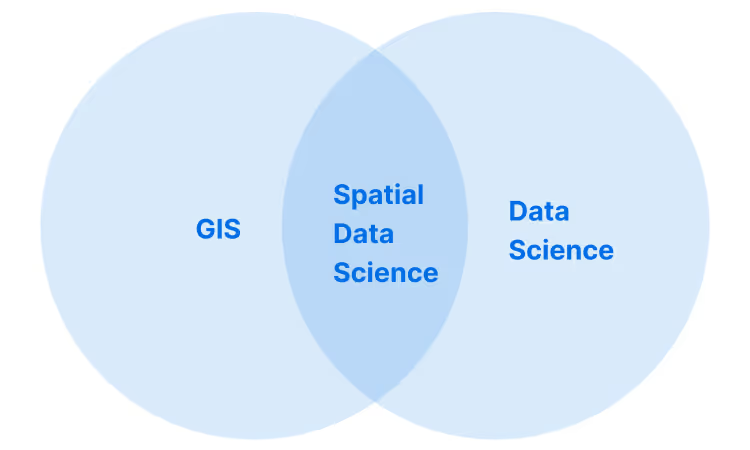
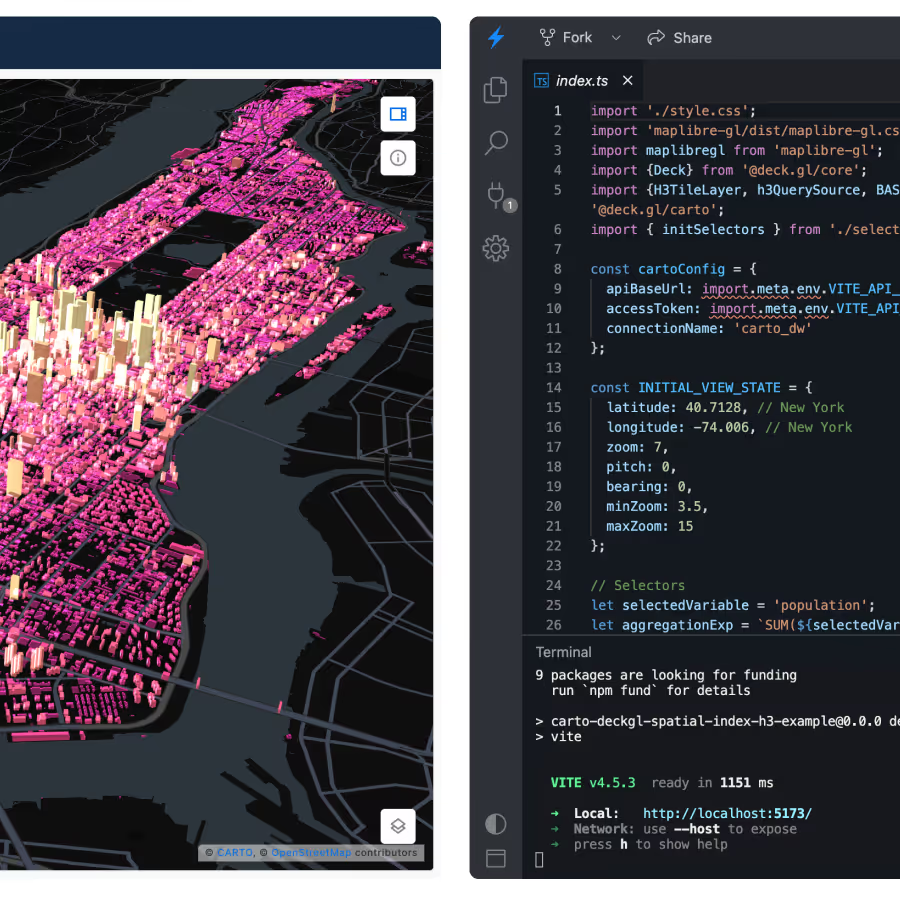
Why is Spatial Data Science important in business?
Private & public sector organizations will be increasing their investment in SDS in the next 2 years (according to The State of Spatial Data Science report).From Retail & Real Estate, to Telecoms & Utilities - Data Science & Analytics leaders are looking to attract expertise in spatial analysis, as well as equipping them with new technology & data streams to enable key use cases that help them to use more spatial insights in their decision making.
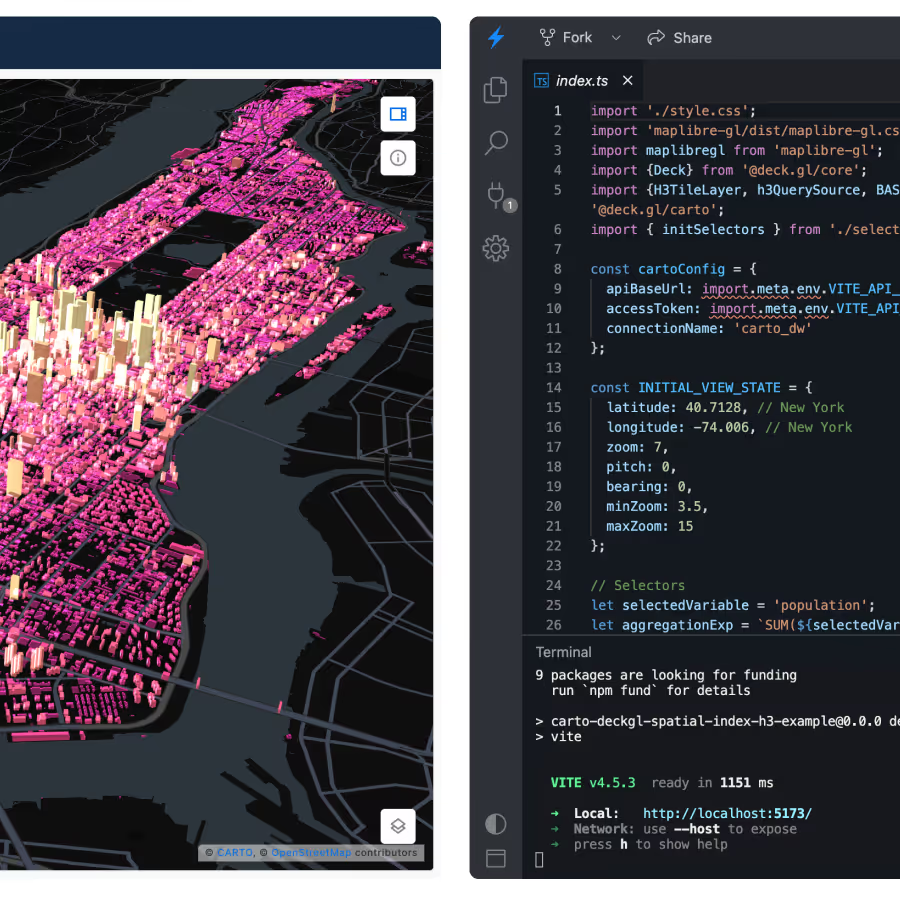
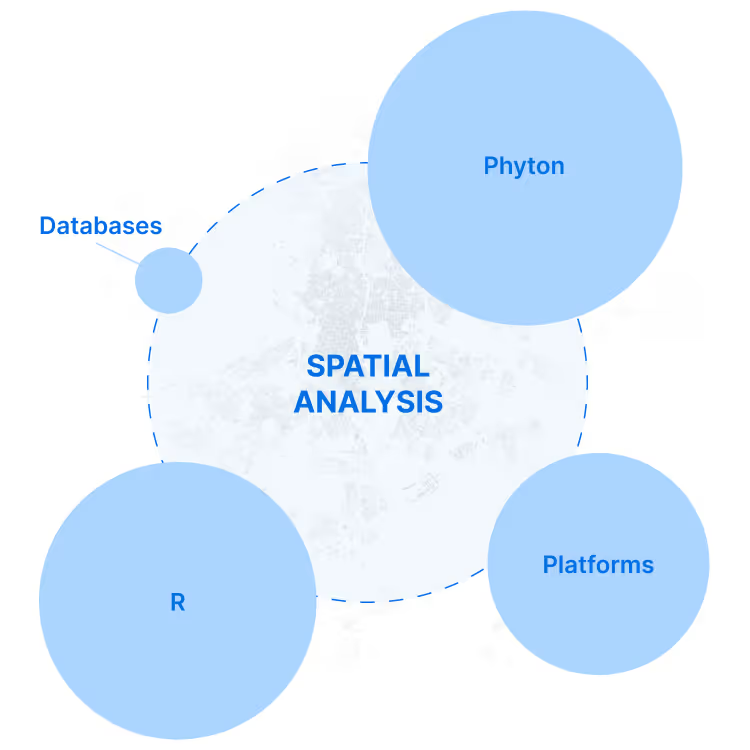
What skills & technologies are used in Spatial Data Science?
Python & R are the most commonly used programming languages in the community. Python’s main libraries for Data Science are well known for being better centralized and organized, but some within the community say that R still has a more complete offering for specific geospatial libraries (vs Data Science more generally). Other key tools include Geopandas, QGIS, PostGIS, CARTOframes and Esri - but, for a full guide we recommend this list of Open Source Spatial Analysis tools.
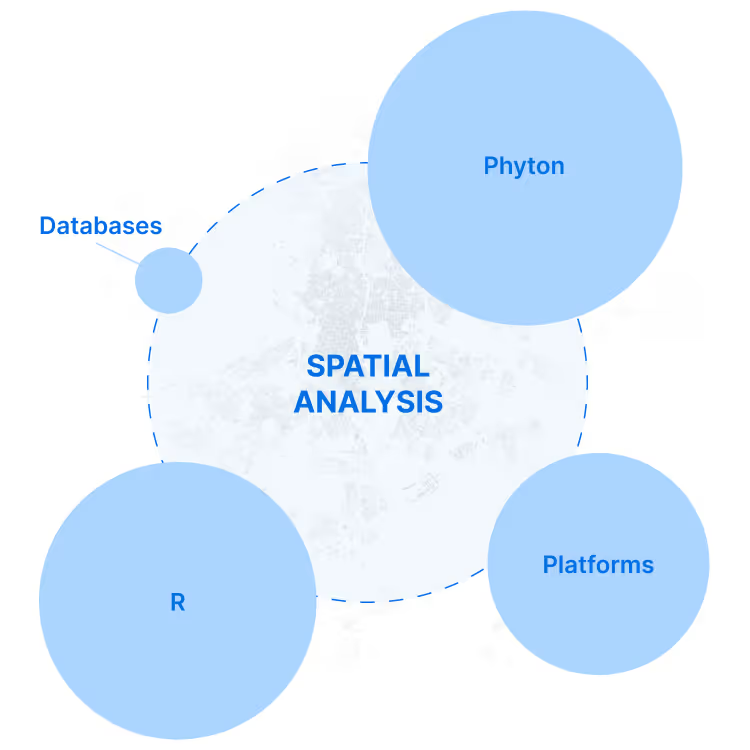
What types of data do Spatial Data Scientists use?
As well as using internal data from their organizations (such as CRM, loyalty card, ecommerce, deliveries, call detail records), they also regularly gather publically available Open Data to enrich their analysis. We also see more & more premium spatial data streams such as Financial, Human Mobility (otherwise known as Foot Traffic), Road Traffic, Points of Interest, Weather, Climate & Housing being used to create more sophisticated spatial models.
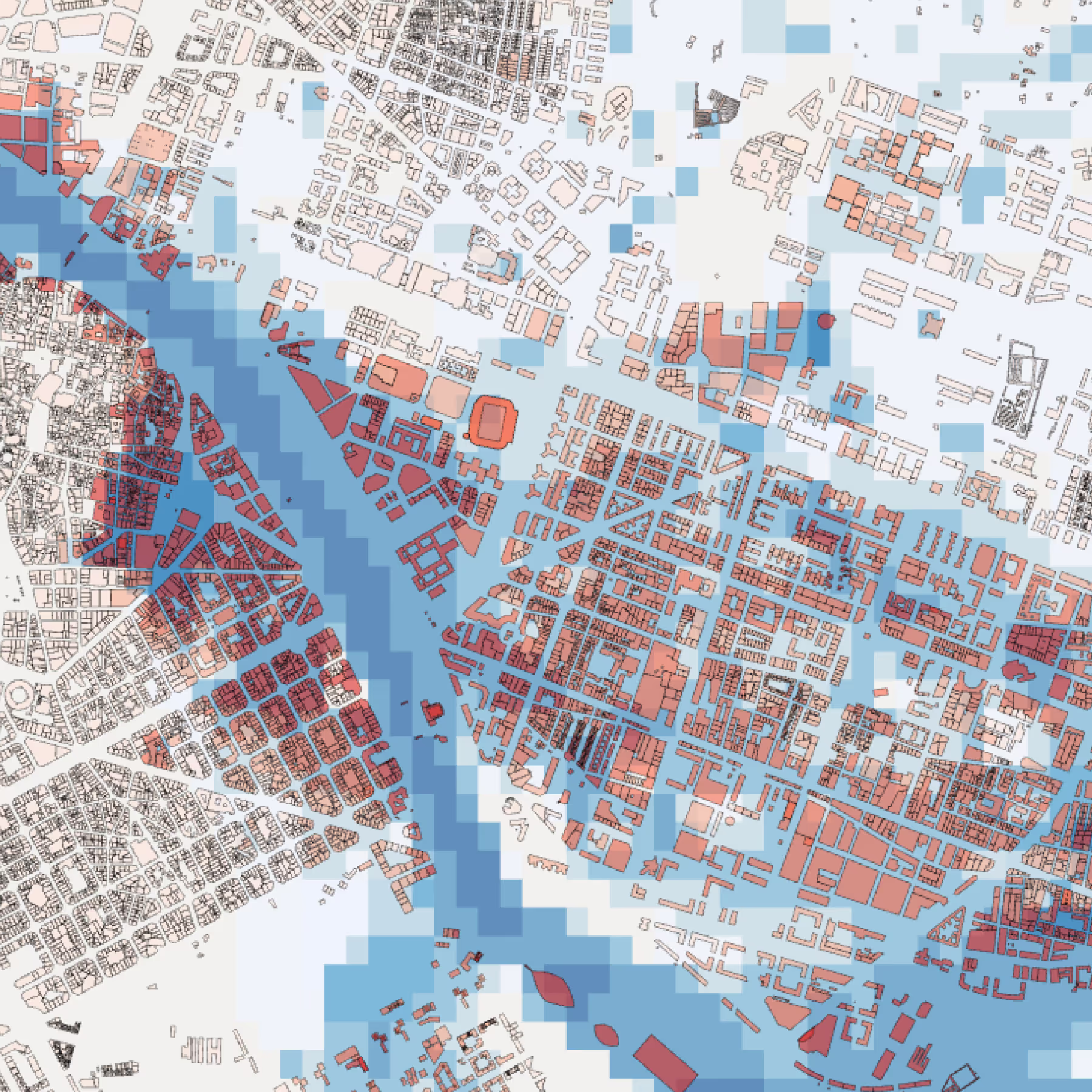
How is it different from GIS?
Geographic information systems (GIS) applies to a wide range of users & use cases, yet is one of those strange anomalies that, despite its value spanning many industries, has remained a niche field - often siloed from other business units. GIS typically refers to varied types of information systems such as websites, apps, or databases that store different types of spatial data.
With new types of users such as Data Scientists, GIS is starting to happen more outside of traditional GIS tools - allowing more sophisticated spatial analyses to take place in connection with new Data Science & Big Data solutions. This shift is allowing Spatial Data Science to emerge as a discipline with greater interactivity with Open Source & Cloud technologies.