Top 3 Insights from The State of Spatial Data Science 2020
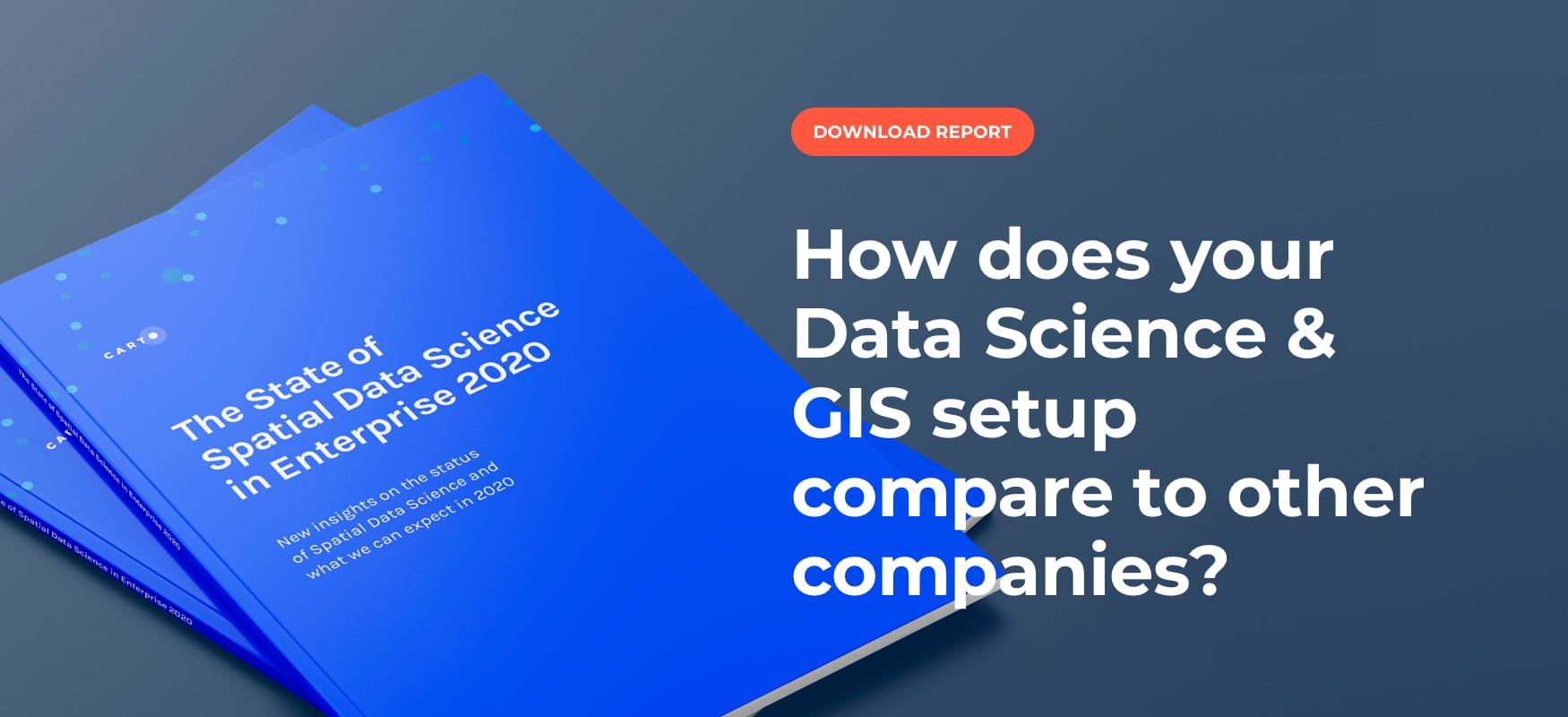
Spatial Data Science is a rapidly growing field and it continues to expand its reach as leaders across dozens of industries recognize its value. In order to understand the trends and shifts for Spatial Data Science across these industries we invited a group of thought leaders from enterprise organizations across the globe to participate in the State of Spatial Data Science in Enterprise 2020 Survey to gain new insights on the status quo and to explore what we can expect in 2020.
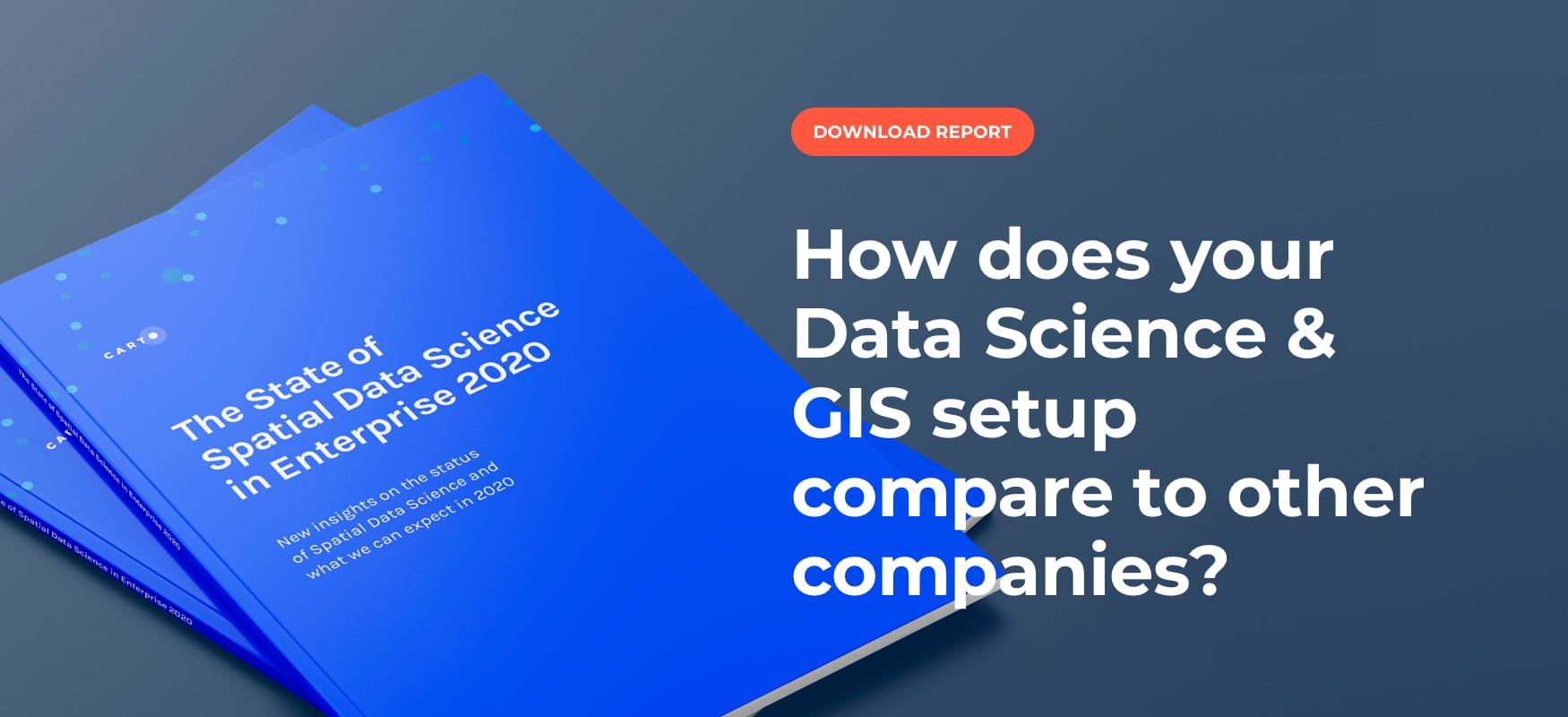
In addition to clicking the image above to access the complete report here are three of the top insights uncovered by the survey!
Spatial and GIS - Growing Less Siloed But Still a Challenge
Our report found that more and more enterprises today are maintaining Data Science and GIS departments. And until spatial skills further proliferate among professional data scientists (a challenge we will discuss below) this is very useful.
Unfortunately collaboration between these departments is no guarantee. While we were very encouraged by the 45% of enterprises that say their DS and GIS teams are daily collaborators that leaves 55% where that is not the case:

Unfortunately when these teams are siloed from one another enterprises are not deriving the maximum value from their data. Organizations need to start to break down these silos in order to advance their Spatial Data Science strategy and tackle spatial problems more holistically.
Python for Spatial Leads R Rises
Coke or Pepsi. Deep Dish or New York Style. Star Wars or Star Trek.
The discussion over the preferred language for Data Science work between Python and R belongs in the pantheon of great debates. And as had been detailed across dozens of think pieces there are a lot of opinions.
So it is no surprise that among survey respondents opinions are split on which language is better for Spatial Data Science operations:

While Python edges out R there were advantages identified for both. Python fans identified a more centralized and organized ecosystem of packages as a key differentiator. Meanwhile proponents of R noted that the language has a more complete offer in terms of specific libraries for Spatial Data Science specifically vs. Data Science more generally.
Spatial Data Science Resources are Rare and In Demand
The field of Spatial Data Science is still in its infancy. It is therefore no surprise that finding the resources necessary to fully access the benefits of the field is a major challenge. Respondents to the survey noted this difficulty not just around acquiring the proper software but also for finding qualified talent to full Spatial Data Science roles.

The full report details the breadth of skills that employers are looking for in candidates for these positions and it is a challenging profile to hire for. That said more and more academic and professional training programs are popping up to raise a new generation of spatially skilled data scientists and to upskill current Data Science professionals. We can anticipate that this talent gap will start to be filled in the coming years. We recently detailed some of these top academic programs and outlined a number of critical SDS skills in our free eBook Becoming a Spatial Data Scientist.
Spatial Data Science is a field growing in scope and importance. But we are still learning a lot about how it is being implemented. Download the full report "The State of Spatial Data Science in Enterprise 2020 " to see all of our findings and insights.
Want to join the discussion about The State of Spatial report Register for the upcoming webinar!