How BBVA is Understanding Cities by Analyzing Credit Card Data

Administrative municipal boundaries such as districts and neighborhoods are often used to describe the behavior of citizens that live within cities. However in most countries people interact within cities without constraining their activity to district or zip code boundary constraints.
So why do so many data visualizations and behavioral explanations of cities rely on administrative divisions that rarely change?
The Urban Analytics initiative at BBVA Data & Analytics in partnership with CARTO presents a new data story to represent city-wide behavior better.
Click here to view the data story.
Using anonymized credit card data they identified functional areas in Madrid Barcelona and Mexico City described city dynamics and compared areas from different cities to gain valuable insights for urban management of public and private services.
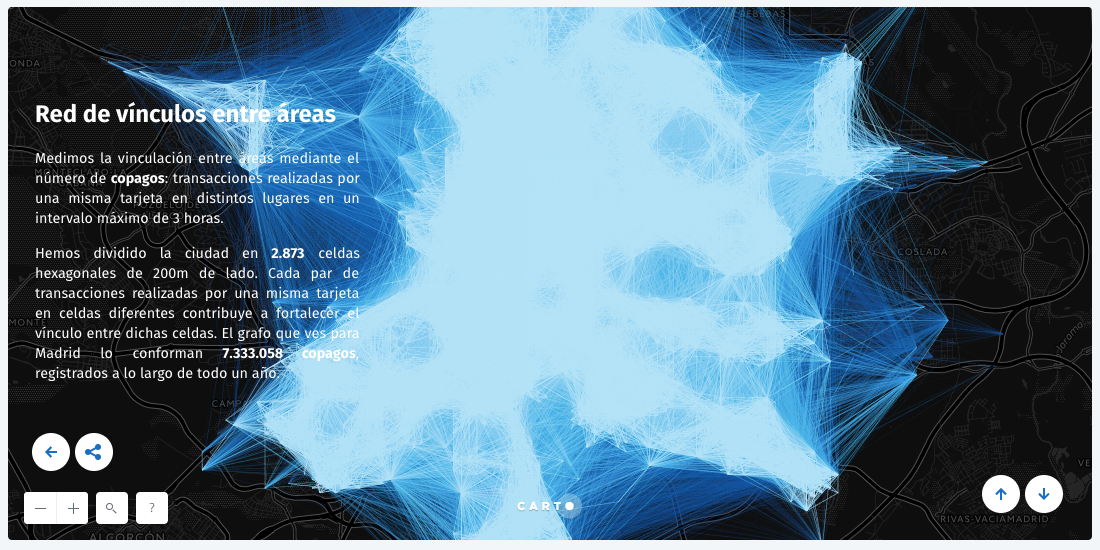
Creating a Network of Connections
The first objective of this project was to establish relationships between different areas of the city using card transactions.
In this case BBVA chose to describe economic mobility patterns through businesses that shared customers.
- First every city was divided in 200-metres-side hexagonal cells.
- They established a maximum time interval of 3 hours to see if the same card had been used in different cells and every time this happened we created a link between those cells.
- They applied this method during a 12-month period ensured that seasonal trends were captured.
BBVA calls these links payment duos and below you can see the co-payment networks obtained for the cities of Madrid Barcelona and CDMX. Creating the network of connections was the foundation for identifying polygons (areas) in the cities that they analyzed.
Identifying Functional Areas with Spatial Analysis and Clustering
BBVA wanted to find a new way of dividing the three cities using this data. They applied community detection algorithms to the three networks to try to find comparisons between the cities.
By using a similar methodology in all three locations they created both clusters of macro-communities and communities essentially both large and small clusters that shared similarities between cities.
These clusters were the basis for finding geographically-constrained commonalities between different groups of people. This method can be applied to any large dataset commercial activity or otherwise.
(The shapefiles containing the macro-communities and communities for each city are available to download in the data story).
Describing Communities Through Common Attributes
The next step was to identify the different common attributes of each community.
From their data BBVA found more than 25 variables associated with three agents: the merchants the clients and the timestamp of the transactions.The last step of the characterization process was to combine these variables to create the 17 attributes of consumption used in their Urban Discovery tool.
For example:
- the attribute Culture doesn’t only refer to communities where there are museums and art galleries but also to a community that attracts clients that spend more than the average in that kind of places.
- Residential Area describes areas with low transaction activity during working hours nonexistent activity of tourists and a low number of merchants.
Other attributes were determined by mixing variables in the data.
All of this careful spatial data analysis results in an interactive tool that allows users to select which attributes they are interested in and see which communities have them.
Launching The Urban Discovery Tool
Anybody visiting or living in a large city will rarely take it all in: we end up scaling it down to a limited area based on where we live work study or where we spend our free time or engage in leisure activities.
The Urban Discovery initiative sets forth an innovative approach to analyzing city dynamics with Location Intelligence identifying functional areas through anonymized data from credit card transactions to visualize and to compare activities in different areas that are not tied to traditional city boundaries.
This tool has many potential applications that can change the way cities and enterprise businesses function:
- Citizen Services. Local governments exist to serve citizens and citizens expect the same innovation from their governments as they see in society around them. More often than not this means using location information to transform they way they deliver service and demonstrate their performance.
- IoT. Cities and governments globally are racing to make better use of actionable data when making decisions. Because so many government activities are inherently location-centric accessing and understanding location-related data is critical. Citizens and stakeholders demand it and agencies are being held accountable to the data they manage.
- Fleeting Routing & Tracking. Businesses are figuring out how to optimize their fleet’s performance and routing.
- Retail and Site Planning. Determining where to put your next store or market your next campaign can make or break your campaign. Using data from Urban Discovery can help make those campaigns a success.
To discuss the initiative further join our webinar with Juan de Dios Romero and Juan Murillo from BBVA Data & Analytics and Isabel Garate Partner and Project Manager at CARTO who will share: