Using Location Data to Identify Communities in Williamsburg, NY
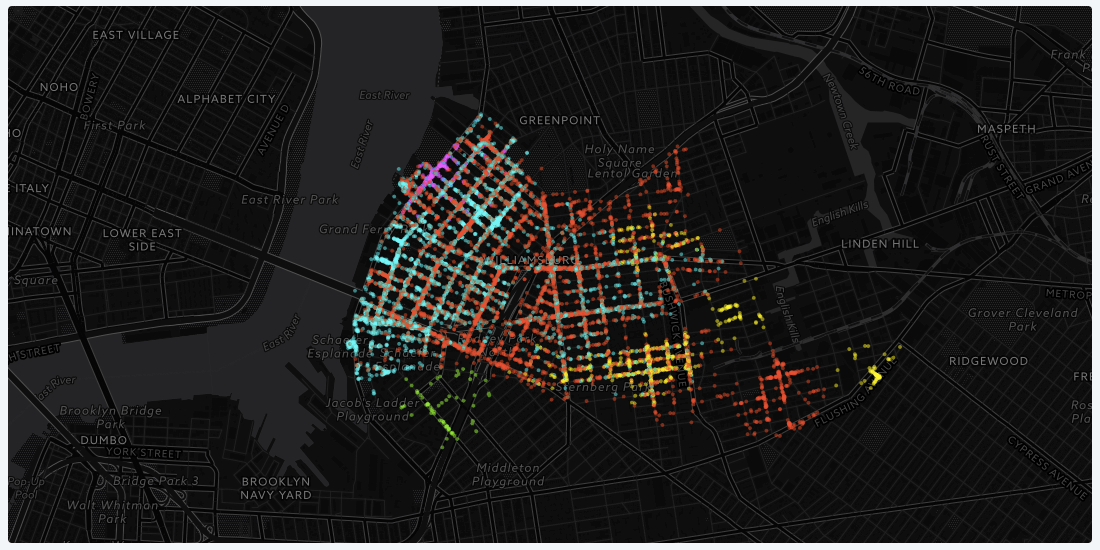
Communities are incredibly difficult to map and most research packs them into isolated groups.
But we know that communities are almost never distinct spatially isolated groups especially when it comes to urban areas.
The same space or area may serve many different groups of people who access different aspects of that space and certain communities can span beyond hard borders like zip codes and census-defined city borders.
With the growth in urban mobility and location data strategies around spatial planning are increasingly addressing the notion that space and land use can be dynamic and flexible changing shape and purpose at different times of the day.
We wanted to explore how we can use data to better understand and define communities of people going beyond spatial borders like zip code and neighborhood boundaries.
We do this through the lens of one neighborhood: Williamsburg New York.
A brief history of Williamsburg NY
Williamsburg has a history of being home to a diverse range of immigrant ethnic communities including Italians and eastern Europeans in the early 20th century refugee or migrant Jewish people during World War II and Hispanics and Puerto Ricans in the 1960s in search of factory jobs. Since the 1970s it has also been a hub for the cultural community as the decline of heavy industry in the area eventually brought an artist and musicians in the area in search of cheap rent and spacious accommodations. And artists it is generally conceived are often the harbingers of gentrification for previously low-rent inner-city neighborhoods.
The density and diversity of Williamsburg has often led to the spatial and cultural territory conflicts ranging from tensions between the Hasidic Jewish community and Hispanic and black minorities in the neighborhood to those between hipsters and poser hipsters.
Add to this an increasing number of tourists and inter-borough tourists to Williamsburg and we can see that the borough is indeed diverse in the types of people that live work visit and play here (despite no longer being the predominantly working-class neighborhood it once was).
Analyzing the Location Data
We wanted to better understand the communities within Williamsburg using location data so we decided to revist the New York City Taxi and Limousine Commission’s open data and the clustering algorithm called DBSCAN which looks for clusters that are at least a minimum number of points and a minimum “distance” away from each other. This diagram below illustrates how this type of clustering works.
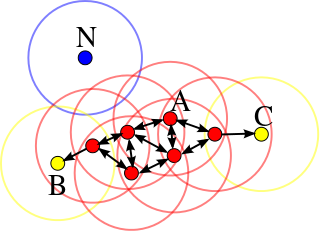
Instead of thinking about distance as a purely spatial concept we wanted to look at the ‘closeness’ of a bundle of characteristics some of which are non-spatial such as the time of the taxi drop-off to find groupings of taxi rides that are similar to each other. The characteristics we clustered are: pick-up and drop-off locationsthe day of the weekthe time of the daythe trip distance
Technical Stuff
For the data-curious readers out there this was my process for creating this map:
Typically when we do these types of clustering analyses we want to first ‘essentialize’ the data by using a dimensionality reduction method such as principal component analysis (PCA) or linear discriminant analysis (LDA) on our features. In this particular case however since we have only 7 features and none of our eigenvalues (or ‘explained’ variances) from our PCA were very big we decided to skip this step and use the original features normalized by their mean and standard deviations. From there we let our DBSCAN algorithm cluster for points that are within at least 0.4 standard deviations “away” from 40 other points using a Euclidean distance as our distance function. In higher dimensions typically 9 or higher Euclidean distances are no longer great metrics as points become essentially uniformly distance from one another.
Find out how city planners around the world are solving traffic congestions with location data in our on-demand webinar "Unlocking Traffic and Commuting Insights with LI"
Identifying the Communities
Using this advanced spatial analysis combined with open location data we’re better able to understand the Williamsburg neighborhood and the communities that exist within it. With this cluster analysis we identified 75 communities five of which we have highlighted here as “partiers” “intra-borough residents” “working class” residents “visitors with expensive taste” and “Orthodox Jewish” residents.
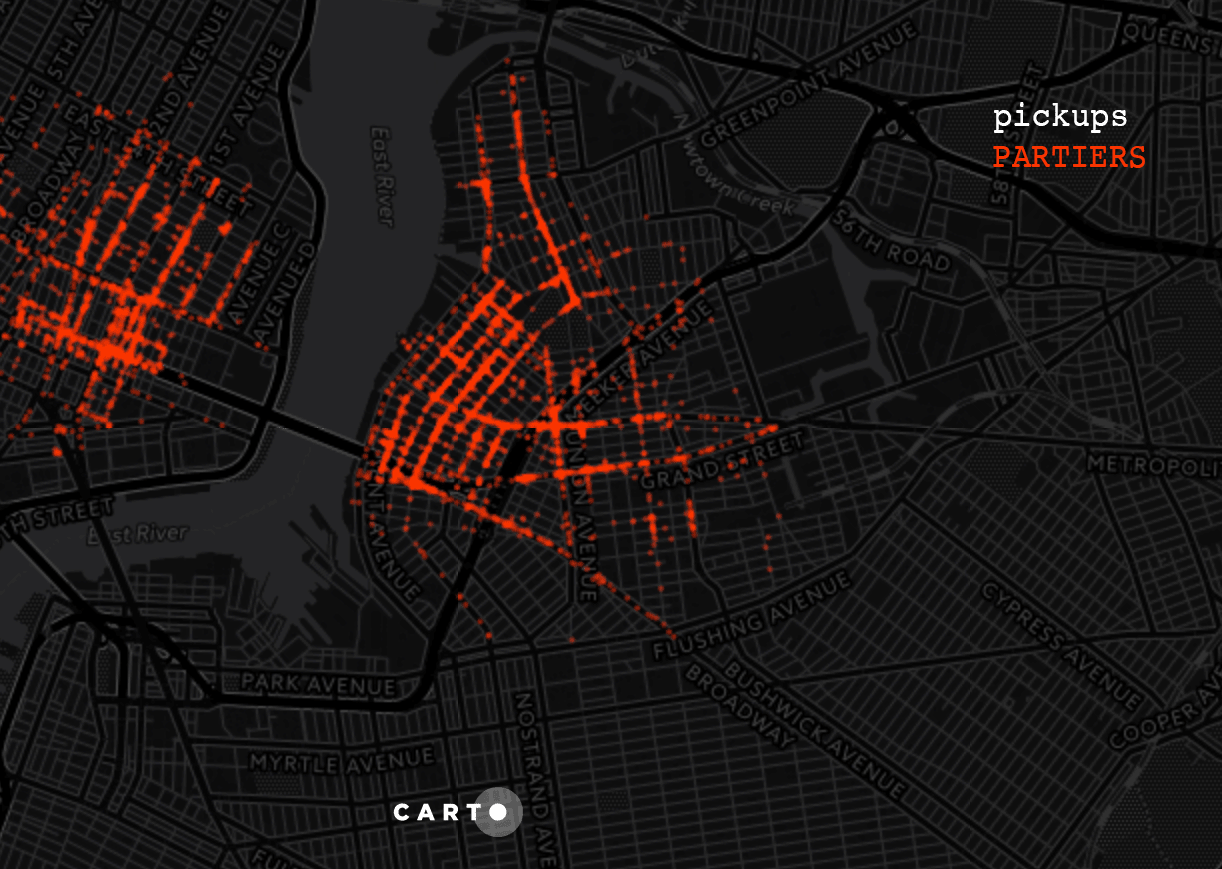
On our map if you toggle for certain groups and times of the day you can see the emergent behavior of these groups: For instance the “partiers” take taxis from Lower Manhattan and Brooklyn to Williamsburg generally pretty late at night the Orthodox Jews do not travel very far and mostly congregate in South Williamsburg.
There are many different ways to label and interpret the data used to create the map but our goal was to highlight an interesting method to investigate communities that occupy similar space. We hope that this map also does justice in representing the beauty of all the many diverse groups of people that visit and live in Williamsburg.