How to Use Spatial Data to Create a Wildfire Risk Map
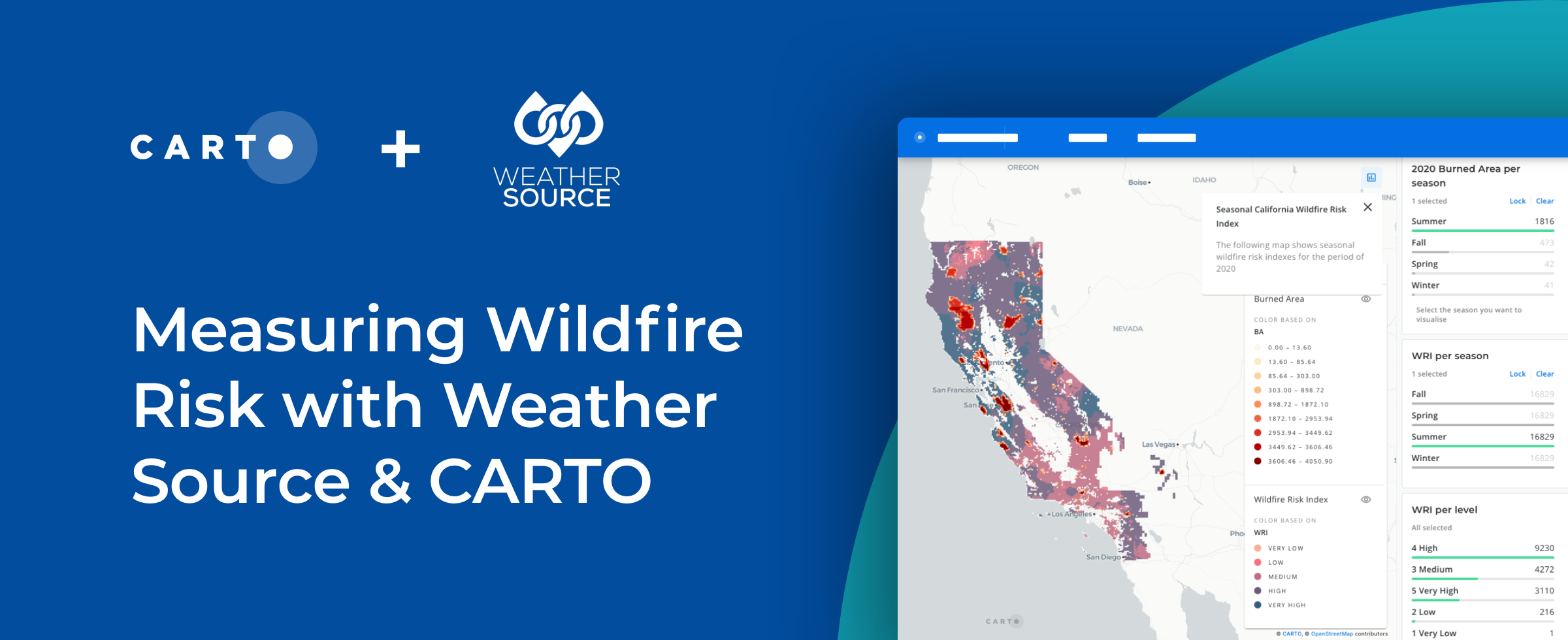
Wildfires can be detrimental to urban and rural areas, causing impacts in the form of injury, death and property loss. In fact, recent studies (here and here) have shown a dramatic increase in global wildfire activity as a result of increasing temperatures, drought and fuel aridity among others, leading to warmer springs, longer summer dry seasons and drier vegetation.
In this context, developing an index to evaluate the potential risk of wildfire ignition is of great importance in several decision-making processes involving public administrations, insurance companies or real estate/investment companies. For instance, a Wildfire Risk Index could be used:
- By government agencies to develop wildfire suppression plans, direct resources to higher risk areas, and aid in emitting wildfire hazardalerts when needed
- By local landscape managers to prioritize and allocate investments to treat wildland fuels and identify areas with a high estimated loss
- By insurance businesses to provide affordable coverage, reduce wildfire risk to communities, and manage their losses, which also gives them a competitive advantage by offering wildfire insurance while others choose not to
- By power and utilities companies to design contingency plans that guarantee energy supply during disaster recovery to critical facilities and vulnerable neighbors
Measuring Wildfire Risk with Weather Source & CARTO
In this blogpost, in partnership with Weather Source, we examined the fires occurring in the state of California for the 2001-2020 period with the aim of determining a spatial Wildfire Risk Index (WRI) that allows decision makers to assess the risk of potential fire occurrences in a certain area. The following map shows the distribution of fires in the California region by year and season, with each dot representing a fire with size proportional to its burned area (BA).
It’s interesting to see that many fires have an unknown cause, while others have been associated with lightning and several human activities (like campfires or smoking). Due to the great variety of wildfire origins, estimating fire occurrence is known to be a complicated task, and the human factor plays an important role.
A drastic increase in wildfire activity can be observed in 2020, when the largest fire occurred (August Complex Fire), burning more than 1 million acres. It is important to mention that fire detection technologies have improved in recent years which may have also influenced the presence of any trend.

Further insights can be obtained if we visualize when the wildfires occurred and how large they were. In the following plot each horizontal line represents a wildfire from when ignition started to when it was contained. Also, the higher we move in the y-axis the larger the resulting burned area (in logarithmic scale for visualization purposes). It shows that most fires occur during the summer and fall months when drought conditions and extreme winds become more frequent and vegetation more dry.

Data Sources
We identified different data sources that can influence wildfires and, therefore, may be useful to determine the spatial WRI. We worked with open and premium data the latter provided by Weather Source.
Wildfire Data (2001-2020)
Perimeters of different fires provided by Weather Source (historical data provided by the Wildland Fire Management Research, Development & Application group at the National Interagency Fire Center) containing the start and end of each fire cause of the ignition and burned area (BA) (see map below).
Geographical Data
Datasets from several sources were used to analyze whether some geography-dependent variables would favor fire presence (see map below). These variables can be considered as static over the period of analysis and include:
- Land Cover type (Esri 10-Meter Land Cover - Microsoft Planetary Data Catalog) which represents the physical coverage of the Earth’s surface (i.e. crops trees water…). Previous studies have shown that different land cover types are more prone to fire than others. For instance, fire selectivity is generally higher for shrublands than for annual crops
- Köpper-Geiger (KG) Climate Classification (see the reference paper) which assigns each region to a different climate group based on seasonal precipitation and temperature patterns.
- Population and elevation (Spatial Features - CARTO): do larger fires occur in remote areas where there is almost no population, or in higher areas that experience more sun exposure?
- Kilometers of roads and distance to the closest road (Roads North America - Global Natural Earth): do fires occur near freeways because of smokers throwing cigarettes on the road?
Meteorological data
Time series data provided by Weather Source was used with the aim of analyzing how changing meteorological factors affect the appearance of wildfires over time. Based on previous studies that linked fire occurrence and severity to drought severity the following variables were selected:
- Standardized precipitation evapotranspiration index (SPEI 2001-2020): it is a drought index based on precipitation and potential evapotranspiration being sensitive to changes in evaporation demand. It can be computed at different time scales or accumulation periods. Negative values indicate dry periods, while positive values relate to wet conditions. This data is originally provided in the NOAA Monthly U.S. Climate Gridded Dataset.

- Soil moisture (SM 2014-2020): it represents the water content of the soil and can be measured at different depths below the soil layer. Larger water content may induce the increase in vegetation growth which can later favor ignition.

Lastly we used median income data from the Sociodemographics - American Community Survey dataset from CARTO Data Observatory to discover further insights on how this index can be used by public administrations, insurance companies and Real Estate/investment companies.
Building a Wildfire Risk Index with Weather Data & Spatial Analysis
To develop the WRI, our method requires first clustering the data into different regions to account for differences in climate factors and properties throughout California. Then for each cluster, the most relevant precursors of fires are investigated, following a different approach depending on the nature of the variables (time-dependent vs time-independent). Once the most relevant triggers are selected the index is constructed by combining those through a weighted mean of the selected variables. The index is then categorized into a specific risk level (Very Low - VL Low - L Medium - M High - H or Very High - VH) and estimated for future periods using seasonal forecasts.
For our analysis we made two different indexes following the same aforementioned methodology, each being useful for specific use-cases:
- Annual index: an index constructed to evaluate the risk of ignition during a specific year, which can be used to gain a general picture of fire-prone areas and compare it with the risk for previous years.
- Seasonal index: an index developed to estimate wildfire risk for each season independently, which can be used to compare the risk with previous seasons and allows organizations to plan their management efforts some months ahead.
Now, we compare the estimated annual risk with the wildfires that took place in 2020. It can be seen that during 2020, North California was more prone to fires than South California. Also, none of the areas present a VL risk level, meaning that 2020 was a dryer year compared to the period of 2001-2019, resulting in higher risk. Interactive maps like the one below are ideal for helping users explore such large-scale yet hyper-local data.
On the other hand, if we use the seasonal WRI to focus on fires occurring during Summer, we can see that many fires occur in VH risk-labeled areas. As noted above 2020 was a particularly bad season for California fires with an unprecedented fire occurrence and severity. This is well reflected by the constructed risk index that when compared to the same season in previous years, shows a high to very-high risk in most of the region.
If we select other seasons in the previous map, we can see that for 2020 colder seasons (Winter and Spring) it shows lower risk than previous years, whereas during hot drier seasons (Summer and Fall) the risk of wildfires increases significantly. It is also noticeable that the WRI in Fall appears to be VH in most cases. This occurs because during these months the forecasted 2020 SPEI values are much lower than those used to define the index (2001-2019) indicating that the 2020 Fall season is much drier than average explaining the elevated risk of ignition as can be also seen in these figures:

Using the WRI to Improve Home Insurance
It may be challenging for insurance companies to design appropriate wildfire coverage policies, in areas where the risk of fires is too high or uncertain. Having a reliable WRI provides competitive advantage in assessing wildfire risk as it can aid in defining coverage costs by increasing deductibles or adapting the premium when necessary. This way, insurers incorporating wildfire risk data into their existing product offerings and operational strategies are able to adjust coverages and design specific coverage plans depending on risk level. For instance policies can be designed to ensure resource and personnel distribution assistance in case of wildfire making sure that you have the right number of claims adjusters on the ground to assess damages.
The WRI presented in this post can also be used to design targeted marketing campaigns. For example in the following map we can visualize the top 5% of median income areas in California. Some of these are high wildfire risk areas so the demands of these specific customers may certainly be different from those of other customer segments.
Future Work
As observed, developing an accurate, reliable WRI is a complicated task. Future efforts could be directed toward including other meteorological variables such as temperature precipitation humidity and atmospheric pressure to find further relationships with the observed burned area.
Additionally, using wildfire data with a better temporal resolution that includes not only the duration and overall burned area of wildfires but also their duration would be beneficial to find more accurate precursors of fire ignition. For instance it would be interesting to use the CCI (ESA) MODIS fire data which contains homogenized satellite burned area data and could be useful to expand the analysis to other regions, where wildfires may not occur as much as in California.
Lastly, exploring other ways to combine the temporal information to construct the index could be of great interest. An approach could be to run a regression model to predict the BA using the selected lagged predictors (and potentially accounting for any spatio-temporal autocorrelation) and then predict the BA for the next season as an indicator of fire presence and severity.
Technical Note:
The main analysis was carried out using all available data up to 2019 (training data) while data from 2020 was then used for testing the proposed methodology.
Clustering California Regions
As mentioned above, from a preliminary analysis it highlights that the land cover type affects the presence of wildfires: there are some regions governed by a specific land cover in which fewer fires occurred such as ‘crops’ and others like ‘trees’, where many ignition points can be seen. Therefore we clustered the data according to each land type and performed a separate analysis for each cluster.
In the following analysis some regions were excluded namely:
- Regions corresponding to clusters with a very low fire occurrence: only those clusters where the percentage of the 2001-2019 total burned area (%BA) was larger than 5% were considered (see figure below). These correspond to ‘trees’ and ‘shrub’ land cover types.
- Desert regions (labeled as BW according to the KG Climate Classification): based on this condition, we excluded the Mojave Desert from our analysis which is labeled as ‘shrubs’ in the Land Cover dataset. Desert areas were excluded because of the very limited vegetation that could ignite a fire (indeed almost no fires occurred in this region as seen in this map).
The total area of California covered in this analysis is shown in the map below and you can see that it covers most of the fires that occurred during the period of study (up to 2019).
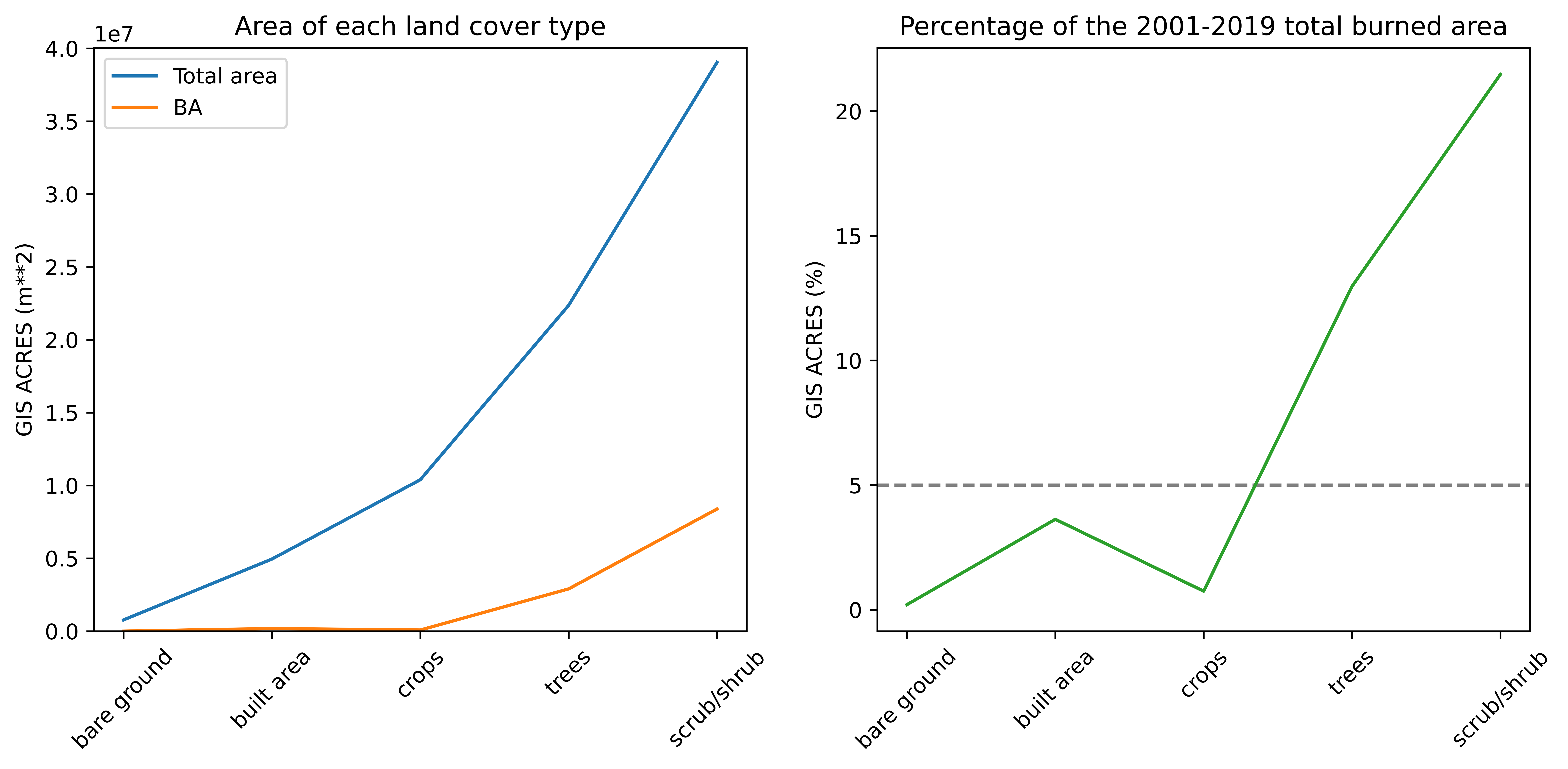
Fire Precursors Selection
For both clusters a preliminary analysis shows that the pairwise correlations between the geographical (static) variables and the burned area for the period of 2001-2019 are all very low as can be seen from the figure below. This suggests that the relevance of such variables on the ignition of fires in these regions is negligible and therefore these variables can be discarded when constructing the risk index.

For selecting the most relevant precursors among meteorological variables which are temporally-dependent we used an approach called Causal Discovery Analysis which detects as many true causal relationships as possible between time series (including their temporal lags) and controls the number of incorrect link detections. For the SPEI 1 3 6 and 12 months were considered; and for the soil moisture , depths of 0.00, 0.01, 0.04, 0.10, 0.30, 0.60, 1.00, 1.60 and 3.00 meters were included. The results of the analysis (see figure below) show that significant negative relationships between the seasonal SPEI and BA time series were found although different accumulation time and lags are selected for each cluster.
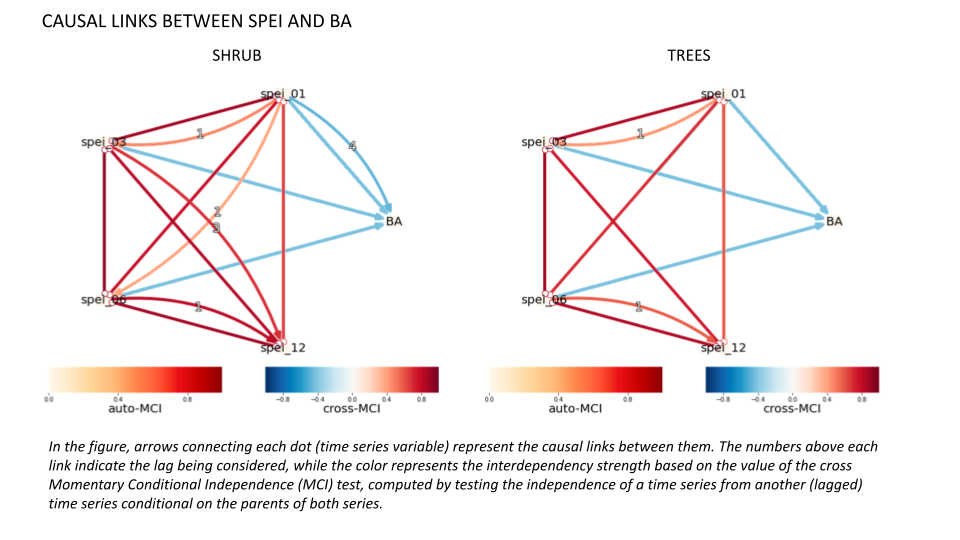
On the other hand no significant causal dependencies were found for the soil moisture variable (and its lags) for either of the clusters, although in this case the result of the analysis might change if data from a longer time period was available.
WRI Definition
Once the fire precursors have been identified we can define how the WRI is calculated. Two different approaches were considered following a similar methodology: (a) an annual index to estimate the risk during a specific year and (b) a seasonal index to evaluate the risk for a particular season.
OPTION A: ANNUAL INDEX - use training data (yearly) to define thresholds for the index levels.
In order to combine the selected SPEI variables to define a wildfire risk index we first computed the weighted mean of these variables for each year where the weights are given as the inverse of the confidence interval value obtained from the Causal Discovery Analysis (the wider the intervals the lower the weight i.e. the less ‘important’ the variable is).
For every time step (year) in the training period (2001-2019) the weighted SPEI index was calculated obtaining for each cluster (shrub and trees) the distribution shown in the figure below. The risk index is then constructed by defining some cut-off points from each distribution: these points were obtained by KMeans clustering which was used to split the data into clusters and define the Wildfire Risk Index based on some risk categories (Very Low - VL, Low - L, Medium - M, High - H, or Very High - VH). Finally, using SPEI data from yearly forecasts (see figure below) and the cut-off points obtained from the distribution of the weighted SPEI index for 2001-2019 we can then compute the WRI for future years (here 2020).
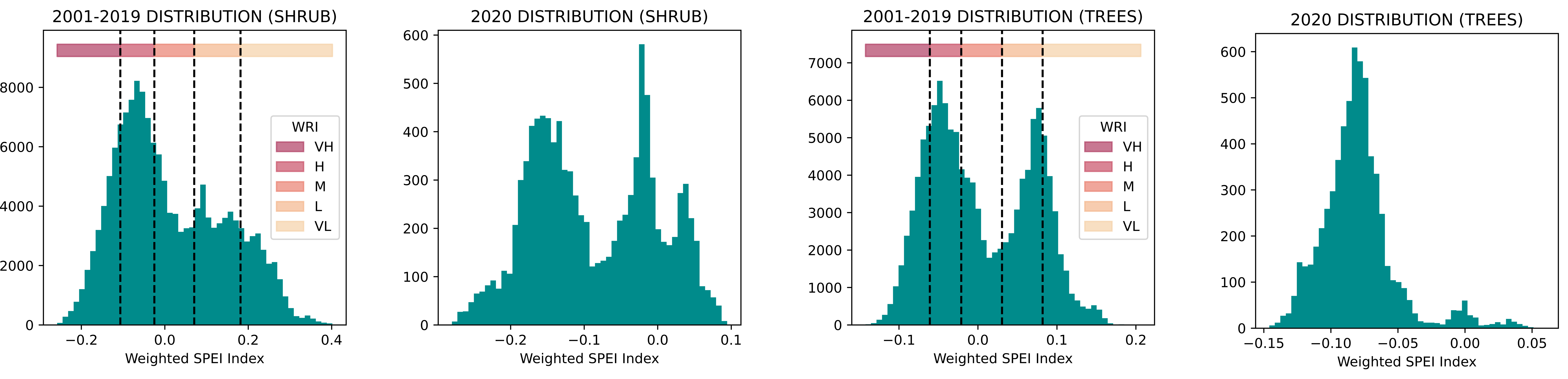
OPTION B: SEASONAL INDEX - use training data (seasonal) to define thresholds for the index levels with different levels depending on the season.
Here to define a wildfire risk index, the weighted mean of the selected SPEI fire precursor variables was computed for each season and year to obtain the weighted SPEI index following the same approach as for the annual WRI calculation. The distributions of each season in the training (2001-2019) and test (2020) periods can be seen below:

As before, the WRI is constructed by defining the cut-off points from each distribution obtaining a total of 4 wildfire risk indexes one for each season of the year. Then we can then compute the WRI for future seasons (here 2020 seasons) as explained previously.

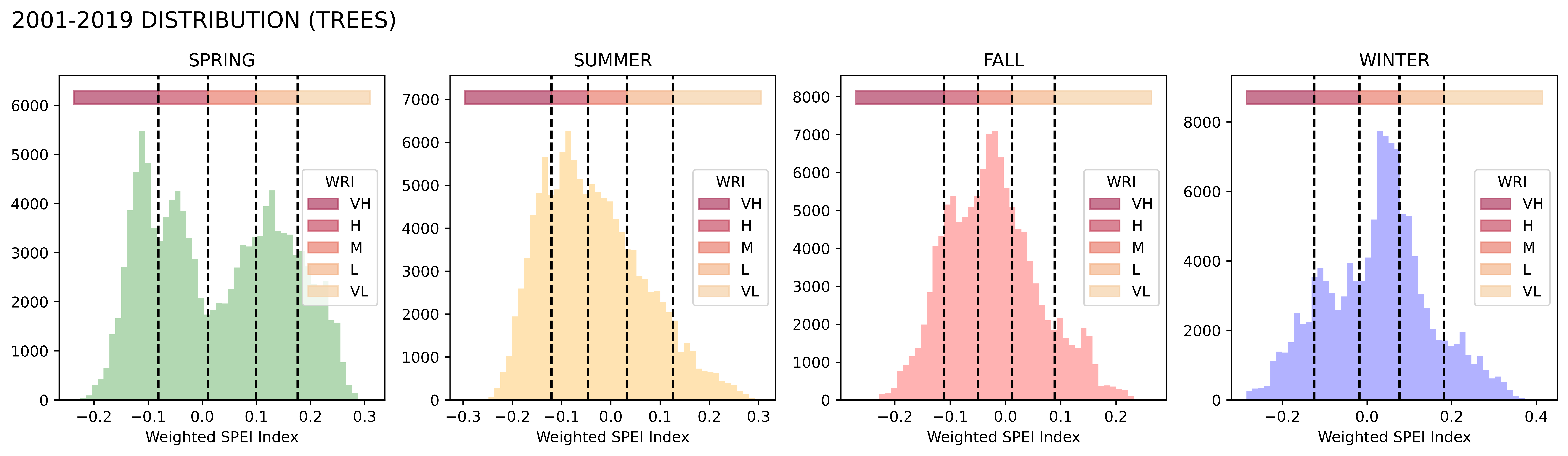