Making Human Mobility Models Fair, Inclusive, & Private

Late in 2020 we announced the creation of CARTO’s Scientific Committee to double down on our efforts to make the work of Spatial Data Scientists more productive and to help spatial analysis continue to improve the world as we know it.
One of the areas of collaboration between CARTO and our Scientific Committee is the organization of scientific workshops to discuss specific issues on Spatial Data Science and propose possible solutions and applications. This June, we held the first scientific workshop “Towards building fair and inclusive human mobility models”.
On June 17th 2021 CARTO’s Data team the members of our Scientific Committee, and their respective teams at MIT, the University of Chicago’s Center for Spatial Data Science University of Liverpool Barcelona Supercomputing Center and Microsoft, joined together to discuss issues involving human mobility data including:
- The representativeness of human mobility data
- Methods for measuring and correcting underlying biases to make models more fair and inclusive
- Methods to improve the usability of the associated data products for both the scientific and business communities.
Additionally, in order to widen the discussion to include insights from industry experts, we drew on the highly valuable participation of members of the Data Science teams at SafeGraph, Unacast, and Vodafone, who also shared their perspectives on these topics.
During this last year, human mobility data has come to prominence due to the COVID-19 pandemic and a lot of research work has been published in areas such as community transmission risks in different contexts effectiveness and impact of social distance policies or analyses of business recovery across sectors and territories.
Prior to the pandemic mobile phone data was already being used in sectors such as urban planning, footfall, census estimates, behavioral science, tourism, and marketing, among others. Although the value of analysing patterns from this type of data are clear (always in an anonymized aggregated and regulatory-compliant manner) there is still a lot of ground to be covered in our understanding of the different biases present in the raw data and how we can adjust for them to ensure the representativeness and fairness of the derived models and insights. Additionally, there is an important and necessary open debate which is still in its early days on how this data should be gathered and used in a way that protects privacy.
Dashboard analysing social distancing metrics during the first wave of the Covid-19 pandemic with data from SafeGraph
Biases in the underlying data can have real and tangible effects on people, companies, and planners. In his presentation, Jamie Saxon of the University of Chicago reviewed some potential practical impacts of data bias as illustrated below. For example policies related to reducing the risk of COVID transmission have typically relied on human mobility data to estimate occupancy and associated risk. However it is often unclear how many people each device count in a coffee shop a gym or a park truly represents. Therefore Jamie shared alternative strategies for measuring ground-truth of human activity in parks and roadways.
Location data can always be made more reliable and representative through multiple data streams. Though often costly, it is critical for the location data community to continue to develop and integrate multiple privacy-preserving measures of activity.

Threats due to biases by Jamie Saxon post-doctoral researcher at University of Chicago
In this context human mobility data refers to data built by analysing the location of groups of mobile handheld devices across space and time. Typically this is achieved by looking at location events that are either measured for a telecommunication company’s cell network or captured by mobile apps. By default the raw data are obtained in ways that have well-known intrinsic biases. In his presentation Prof. Esteban Moro from MIT and UC3M reviewed the multiple biases that need to be accounted for when analysing location-based service (LBS) data, as illustrated in the image below. These biases span four dimensions:
- Demographic: the raw sample may overrepresent certain groups of people
- Geographic: mobile phone penetration and usage is not the same in rural versus urban communities and across different countries
- Temporal: the devices represented in the sample vary over time
- Behavioral: only certain apps measure location events.
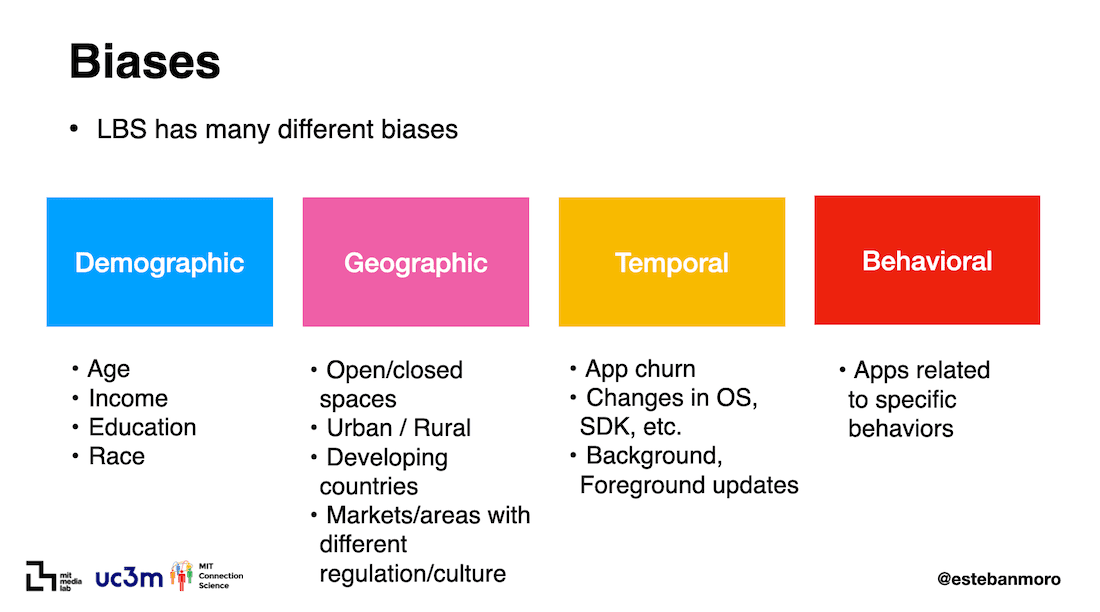
Biases in human mobility data by Esteban Moro researcher and professor at MIT and UC3M
All industry participants agreed that bias compensation is one of their top priorities and something they have already been adjusting for when building their data products. However, given what little is known about the people who make up the sample (by design) and the very limited ground truth data that is available in order to correct biases this still remains an important research challenge to work on.

Considering bias at multiple stages following the Data Science Ethics framework, as presented by Jeff Ho Technical Product Manager at SafeGraph.
Alleviating the biases
During the workshop different approaches for bias alleviation were shared and discussed among academic and industry participants. Examples included:
- Optimizing the panel design looking for representativeness in the sample;
- Pre-stratification defining a panel of users according to specific strata, with the aim to minimize geographical, temporal, and demographic biases. This strategy could be designed to be use-case specific;
- Post-stratification, re-weighting the results based on the different strata. This strategy is in most cases already adopted and made region-specific (i.e. upsampling underrepresented areas or demographic groups based on Census data);
- Machine learning models based on IP clusters identifying app traffic patterns to correct device user age;
- Looking for ground-truth and leveraging complementary data (i.e. payments measured audiences in specific events industrial facilities sensors and computer vision technologies).
It is also important to identify the limits of mobility data and understand which applications it is best suited for to support unbiased decision-making.
Vodafone Analytics
Daily footfall aggregations with Unacast data
Privacy-conscious data collection and use
As adoption of this data for advanced analytics has grown in recent years so have society’s concerns about privacy protection and ethics around the use of this data. New regulations such as the General Data Protection Regulation (GDPR) in Europe and California’s Consumer Privacy Act (CCPA) in the US have emerged and mobile phone manufacturers are implementing new privacy changes in their operating systems. However there is still an open debate on the trade-offs and how to balance the legitimate privacy concerns of individuals and the positive societal value of allowing the use of the data for analytics purposes. As we have seen more clearly during this pandemic, we believe there is still a lot of value in using this data for applications that do good to our society and could allow for better and fairer public policies as well as being an enabler for progress.
In this context several ideas were put on the table for further discussion and investigation:
- Removal of individual device IDs and generation of clustered device IDs based on inclusive segmentation;
- Clearer and easier ways to activate opt-in and opt-out methods including OS-wide opt-out settings for GDPR;
- More incentives for users willing to share the data they generate;
- Changing the hashed IDs on a daily or weekly basis still allowing certain valuable analysis but limiting options to track individual users;
- Development of other anonymous datastreams for context-specific de-biasing using technologies from sensors or edge-based computer vision to aggregate payments.
We are hoping to convene a broader conversation not only between academia and industry players but also with regulators and administrations. Census Bureau data can also serve as a paradigm for how to aggregate individual and sensitive data for analysis. We encourage academic and industry organizations to continue researching key aggregated metrics that will provide the most value to our society and find ways to generate them without compromising the individual right for privacy.
The organisers of the workshop would like to thank Jeff Ho (SafeGraph) Benjamin Thürer (Unacast) Gerardo Lastra (Vodafone) Dan Morris (Microsoft) Alessia Calafiore (University of Liverpool) James Cheshire (University College London) Miguel Álvarez (CARTO) Esteban Moro (MIT and UC3M) James Saxon (University of Chicago) and Alex Singleton (University of Liverpool) for their contributions.
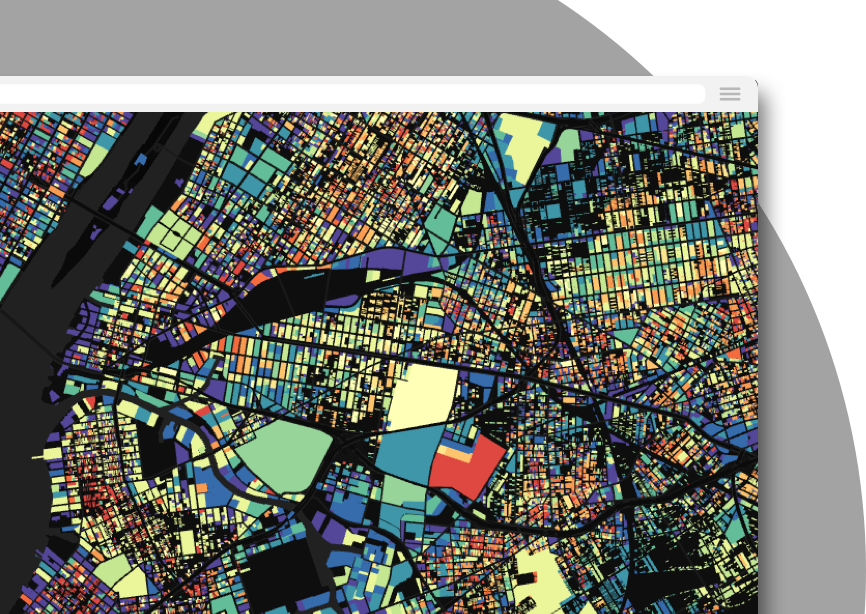
Want to get started? Sign up for a free account