4 Key Points on Raster Analytics from CARTO & Snowflake
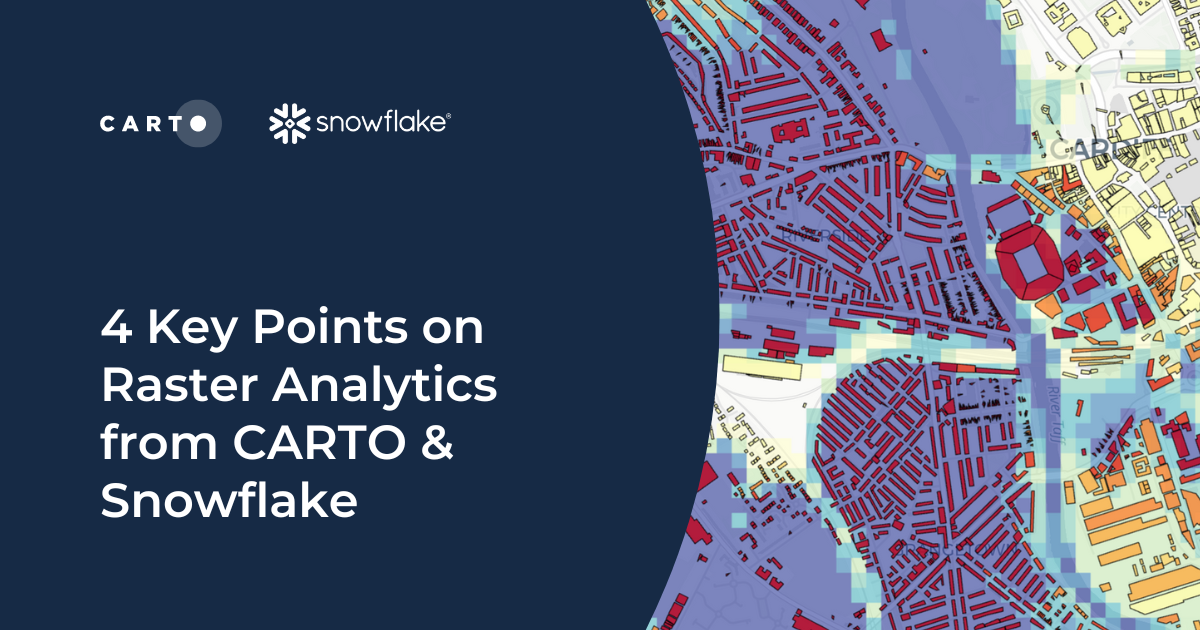
Traditionally, working with large raster datasets using desktop GIS tools has been slow and inefficient due to their size and the processing power required. However, raster analytics have now arrived on the cloud to make managing raster datasets faster and easier!
Recently, we shared new advancements around raster analytic capabilities available in the Snowflake environment. Missed the live session? Watch the on-demand recording here anytime. Below, you’ll find a summary of the key insights that our experts, Jaime Sanchez, Tech lead at CARTO, and Fawad Qureshi, Global Field CTO at Snowflake, discussed.
.webp)
The main point highlighted was the importance of using both raster and vector data for more complete spatial analyses. Raster data provides detailed, pixel-based information, ideal for tasks like environmental monitoring and climate analysis. It is typically used to represent continuous data, such as elevation or land cover. Vector data, on the other hand, offers precise geometric shapes useful for mapping features such as boundaries and infrastructures.
It is a common misconception that one is better than the other (learn more in this article).
Rather than viewing raster and vector as separate entities, it is important to understand each of their strengths and weaknesses and how these, in fact, make them complementary in nature.
For example, if you were trying to adapt your supply chain for EUDR compliance, analyzing raster aerial imagery is ideal for identifying areas of deforestation. However, it’s by integrating this analysis with vector data of farm boundaries that analysts can undertake an actionable analysis of environmental impact.
.gif)
CARTO has developed a way to store and manage rasters as tables so users can query them both using SQL and our no-code tool CARTO Workflows. Here’s how it works! Raster data is partitioned by Quadbin grid; a type of data known as a Spatial Index. In this format, location is encoded through a short reference ID, enabling highly performant storage of big data. The actual raster data itself is stored as a binary array of Quadbins, each containing the raster values. This means the performance of spatial queries on the raster data is significantly improved. Alongside the indexed structure, each raster includes essential metadata detailing the specifics of the raster file, such as its origin, resolution, and format. This structure ensures that all necessary information is readily accessible.
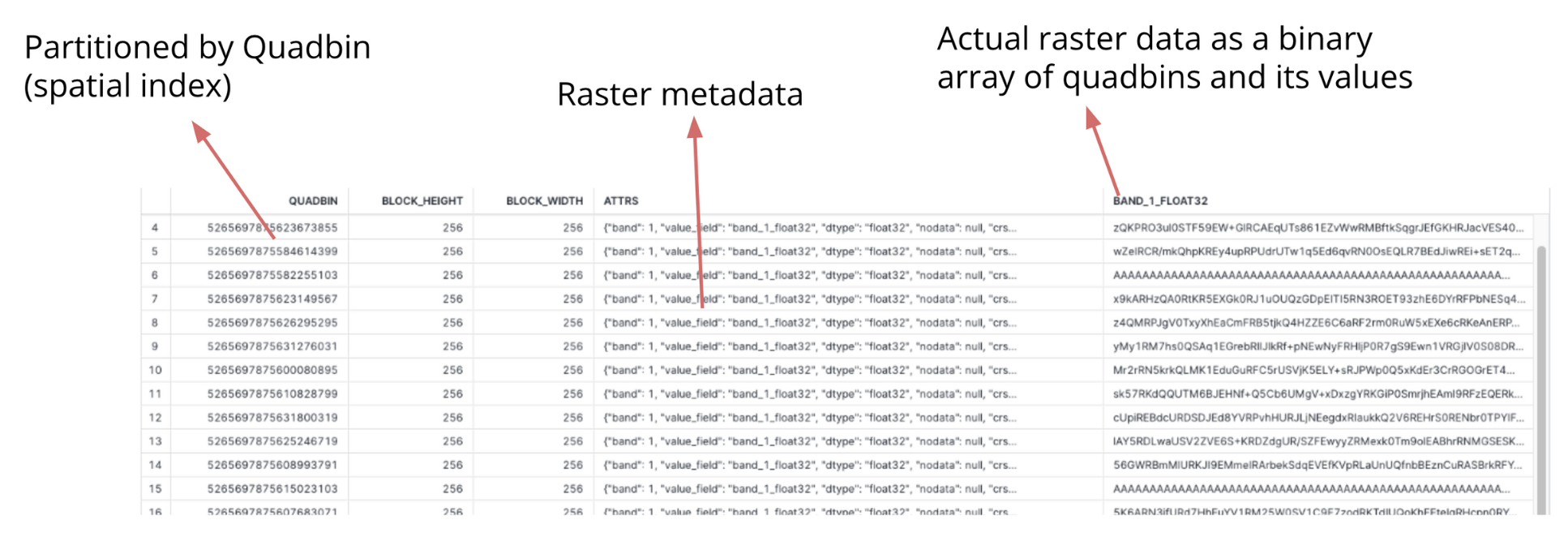
To facilitate more complex analyses, CARTO also provides a suite of user-defined functions (UDFs) that users can access through the Snowflake Native App via Snowpark Container Services. These UDFs allow users to perform sophisticated spatial analyses - such as intersections and joins between vector and raster - directly within Snowflake.
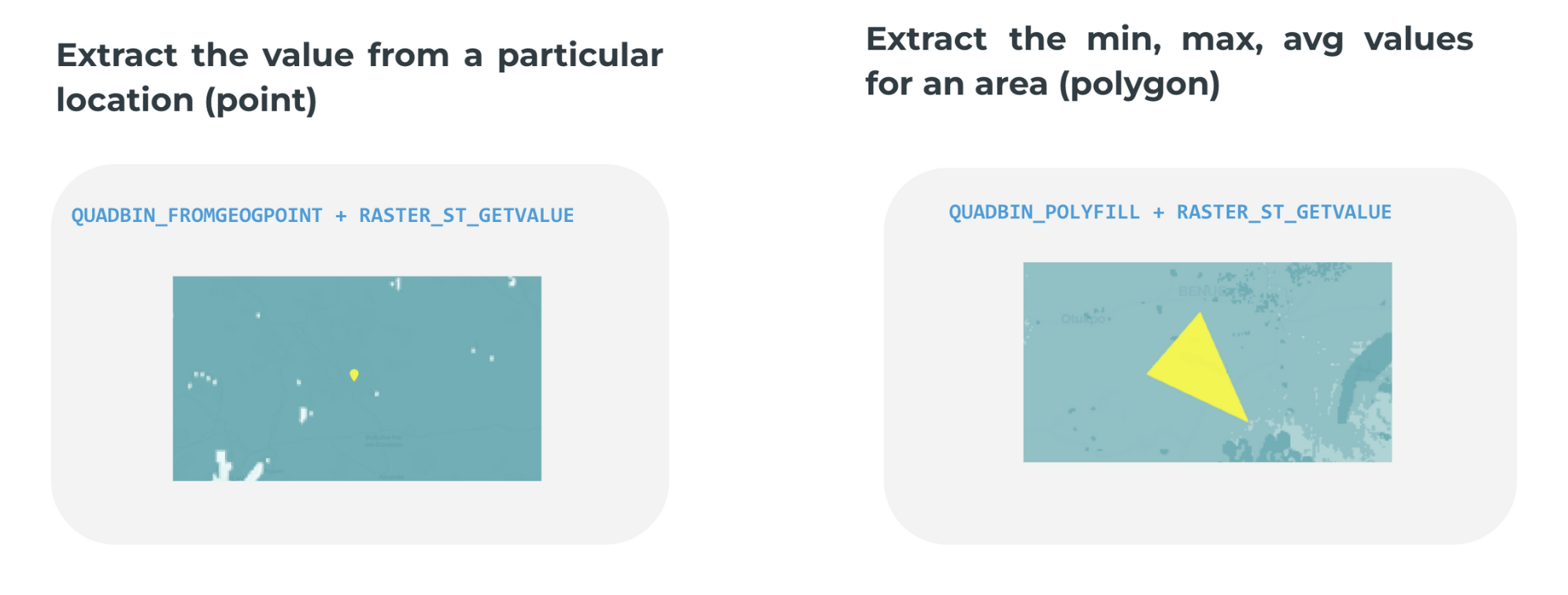
If you’ve ever worked with raster data, you know it can be time-consuming to ETL your data and set up your environment before you can even start analyzing your data.
The CARTO Data Loader helps cloud-optimize raster data before you upload it.
The CARTO Data Loader allows you to convert raster data to a new cloud-optimized raster format designed to streamline the handling and analysis of large raster datasets such as environmental and climate data. Storing rasters as multi-dimensional arrays improves data storage efficiency and speeds up processing.

*Currently, the loader only supports GeoTiff, but it will soon include other data formats like NetCDF.
In addition to raster analytics, Snowflake users can benefit from a wide range of advanced spatial analysis capabilities when deploying CARTO as a Native App. These include access to our Analytics Toolbox for wider advanced data processing, the ability to subscribe to over 12,000 curated geospatial datasets through our Data Observatory, and access to our advanced developer toolkit for seamlessly creating custom applications.

In a nutshell, bringing raster data analytics into the cloud solves many of the challenges that come with analyzing large raster datasets. By using both raster and vector data, and taking advantage of tools like CARTO Workflows and Analytics Toolbox, you can make your spatial analysis more scalable and complete.
Looking to scale up your raster analysis? Request a demo with one of our experts to start your cloud-native spatial journey today!