CARTO for Retail, the Spatial Analytics Platform for Global Retailers

Today we are excited to announce the general availability of CARTO for Retail, a cloud native analytics platform designed specifically for Retailers.
CARTO for Retail addresses the most important data challenges faced by the sector today, as brick-and-mortar retailers realign their property footprint supply operations and marketing efforts in response to ever-evolving consumer behaviors and the return to near-normality.
We work with some of the world’s leading retail brands, including Asda, El Corte Inglés and Carrefour. With this integrated offering, users can now generate valuable location-based insights and tackle the most important strategic decisions in retail, such as where to locate new stores, consolidate their existing physical footprint or optimize their supply chain networks.
A Cloud Native Approach to Retail Analytics
This ground-up development has been designed to run key analytical workloads on the leading cloud data platforms, such as Google BigQuery, Amazon Redshift, Snowflake and Databricks.
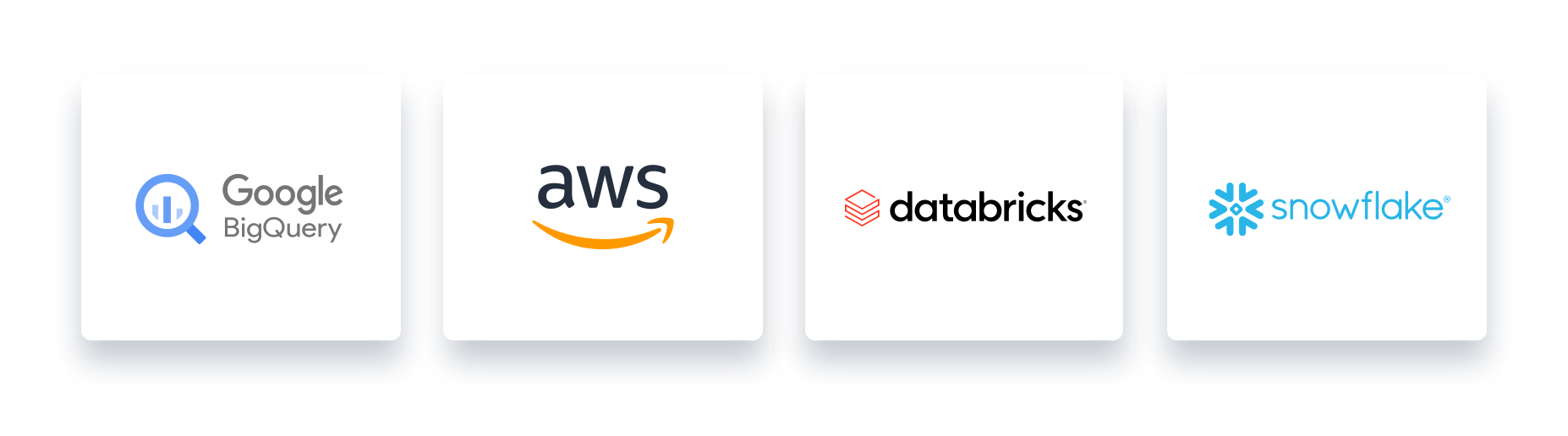
The CARTO platform offers seamless connectivity, so data can be processed and analyzed natively in the cloud, whilst benefiting from the near-limitless scalability that cloud can offer.
In addition, we have developed more than 100 advanced analytical functions, extending the spatial support available in these cloud data warehouses. Data Scientists working in Retail, can now run analytical workloads and models natively in the cloud, and build out powerful dashboards and visualizations to underpin their decisioning processes.
Data Analytics & Retail Specific Apps

Access 1000s of Spatial Datasets
CARTO for Retail brings together a complete suite of retail specific modules, in a single platform. In addition to our leading mapping and analytics tool Builder, the platform also opens up access to more than 11,000 spatial dataset through our Data Observatory. Users can run enrichment processes to deepen their understanding of customers and store locations using Demographic, Point of Interest, Human Mobility and Behavioral datasets from the leading data providers including Experian, Vodafone and TomTom.
The Analytics Toolbox for Retail
Retailers now have access to an unparalleled level of analysis functionality with our embedded analytics module. The Analytics Toolbox for Retail includes some of the most important spatial analysis functions for retail site selection and planning decisions such as Clustering, Commercial Hotspots, Twin Areas, White Space and Store Revenue Prediction analysis. These functions are currently available for Google BigQuery, and can be executed directly from CARTO for Retail or the cloud console itself, using simple SQL commands. In the coming weeks we will be launching similar retail functions for other leading cloud platforms.
Out-of-the-Box Retail Apps & Developer Resources
CARTO for Retail integrates pre-built applications for Retail Location Intelligence. Our Site Selection app, is an end-to-end solution to power retail site evaluation decisions, and integrates map visualization, 3rd party spatial data and a set of ML-driven analytical functions so retailers can quickly and simply assess network expansion opportunities, using an easy-to-use user interface.
For Retailers looking to develop their own visualizations and spatial applications CARTO also unlocks a full suite of developer resources, including APIs and front-end frameworks. For more detailed information, check out our Developer Resources.
With the launch of CARTO for Retail, we continue to evolve our cloud-native spatial offering, so global retailers can access the most advanced location data and analytics to power their strategic decision-making.
If you would like to find out more about CARTO for Retail, sign up for our webinar on the 2nd June, or contact us for a personalized demo of its full capabilities.